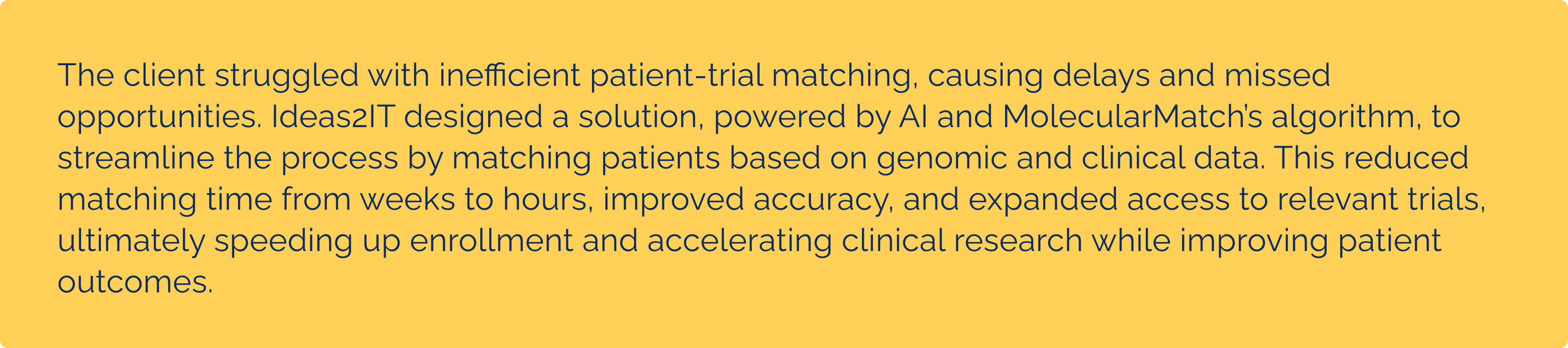
Overcoming Inefficiencies in Clinical Trial Patient Matching
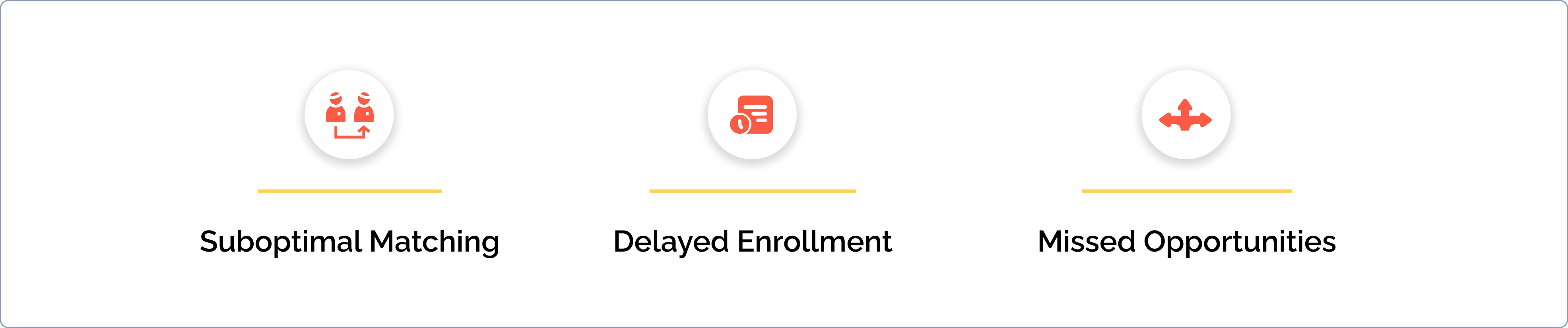
Matching patients to the correct clinical trials has traditionally been inefficient and error-prone, often relying on manual processes and incomplete data. These challenges resulted in missed opportunities, slowing down the trial enrollment process and affecting treatment timelines.The client faced significant challenges in accurately matching patients with suitable clinical trials. Traditional methods could not process large, complex datasets, leading to delays in finding appropriate trials, missed enrollment opportunities, and suboptimal trial recruitment.
AI-Powered Solution for Enhanced Trial Matching Accuracy
The Clinical Trial Match solution was designed by Ideas2it leveraging MolecularMatch, a leader in clinical informatics company with expertise in precision oncology, immunotherapy, and bioinformatics, combined with clinical insights empowering solution. The solution involved the integration of various advanced technologies to provide a seamless, scalable platform for clinical trial matching.
Key technical components included:
- AI Models for Data Inconsistency Detection: The AI models were trained to identify discrepancies across multiple data sources. By cross-referencing trial data against pre-established patterns, historical data, and clinical benchmarks, the system could pinpoint inconsistencies that might have otherwise gone unnoticed. Once identified, the system flagged these inconsistencies for immediate review and resolution.
- Missing Data Identification and Rectification: Missing data is one of the most significant issues in clinical trials, often resulting from incomplete forms, errors in data entry, or patients dropping out. The AI models were designed to not only identify missing data points but also suggest possible values based on statistical patterns, predictive models, and available contextual information. This helped fill gaps in a way that maintained the integrity of the data, ensuring completeness for analysis.
- Anomaly Detection: The AI system employed advanced machine learning algorithms to detect outliers or anomalies in trial data, such as unexpected spikes in measurements or unusual patient outcomes. By analyzing trends over time and comparing them to historical patterns, the system could quickly identify anomalies that might indicate issues like data entry errors or experimental biases.
- Standardization to Regulatory Standards: After detecting and correcting errors, the data was standardized to the CDISC SDTM (Study Data Tabulation Model) standards, which are recognized by regulatory authorities like the FDA and EMA.
Technologies Used
- MolecularMatch Algorithm: A proprietary algorithm that drives precise clinical trial matching by considering genomic and clinical factors.
- AWS SageMaker: Used for model training, data processing, and real-time predictions, enabling the deployment of scalable machine learning models.
- Docker: Containerization of models to ensure scalable and efficient deployment.
- RESTful APIs: For real-time integration with external systems, providing seamless access to trial recommendations.
- Cloud Infrastructure: Powered by AWS, ensuring scalability and real-time data processing capabilities.
Driving Precision, Speed, and Enrollment Efficiency in Clinical Trials
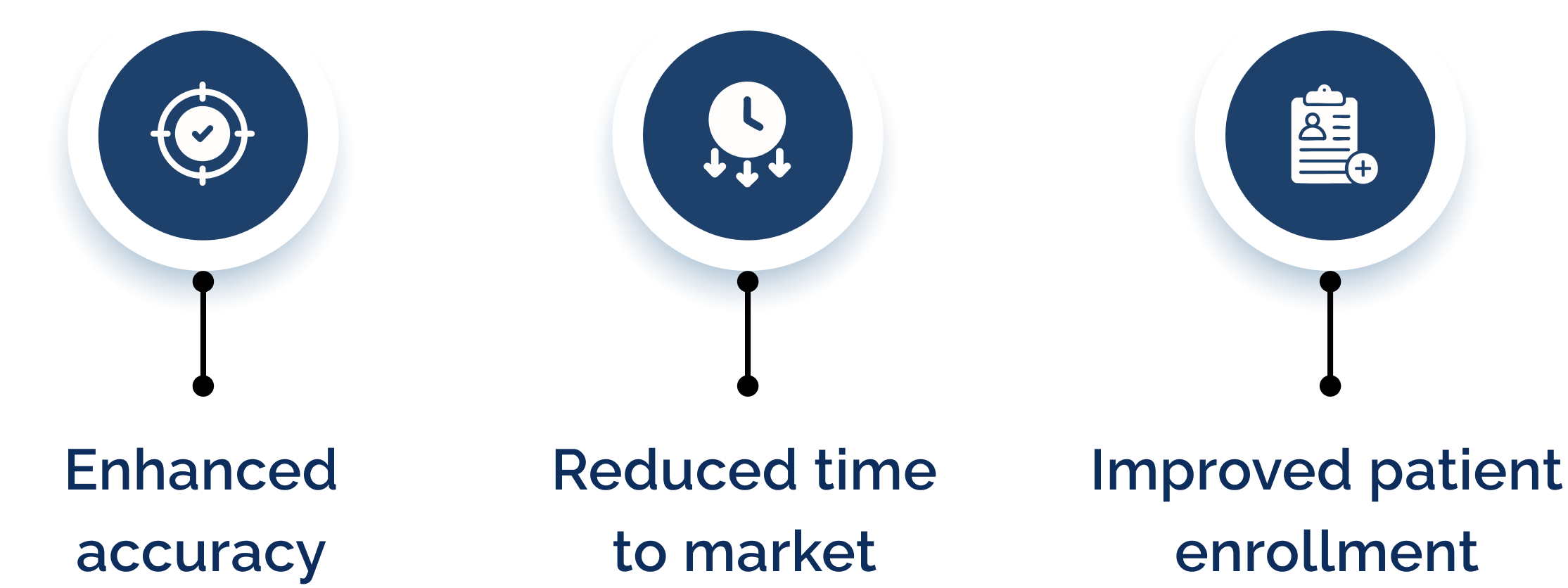
The Clinical Trial Match solution brought about substantial improvements in trial matching accuracy, speed, and patient access:
- The predictive algorithm enhanced the accuracy of matching patients to relevant clinical trials. By processing detailed genomic, clinical, and demographic data, the platform generated more precise trial recommendations, leading to better treatment outcomes for patients.
- The use of real-time machine learning models significantly reduced the time it took to match patients with clinical trials. What previously took 2-4 weeks was now completed in 4 to 8 hours, providing faster, actionable insights to facilitate trial enrollment.
- The integration of genomic and clinical data ensured that patients had access to a broader range of clinical trials, including rare and specialized studies that were previously hard to identify. This expanded access allowed for better treatment options and a higher likelihood of success.
- The solution led to a higher rate of patient enrollment in clinical trials. By streamlining the matching process, more patients were quickly identified for suitable trials, which accelerated research timelines and improved treatment options for patients
The Clinical Trial Match solution successfully transformed the process of matching patients to clinical trials by leveraging predictive analytics, machine learning, and scalable cloud infrastructure. This solution provides better access to life-saving clinical trials.