Preface
Imagine it's 2027—your organization has seamlessly integrated Generative AI (Gen AI) into its core operations, transforming innovation, growth, and customer engagement. You’ve moved beyond the initial hype to establish your enterprise as a leader in leveraging AI for tangible value.
This journey required strategic foresight, navigating regulatory landscapes, and overcoming early skepticism.
Rewind to 2024. The Enterprise Gen AI landscape has reached a critical juncture. Reports suggest that the Gen AI market size is expected to exceed $66 billion by the end of 2024 and an anticipated yearly growth rate of 20.8% (CAGR 2024-2030). As Gen AI became a top executive priority, you faced the challenge of capturing its value sustainably and at scale.
While you appreciated the unprecedented potential impact of Gen AI solutions, you recognized that achieving significant business value meant going beyond novelty use cases and maneuvering current Gen AI challenges and risks.
Our research shows that while numerous use cases span every industry, identifying the most valuable "Gen AI native use cases" that maximize business value while meeting enterprise standards for privacy, data governance, and security amidst a rapidly evolving tool landscape is a significant challenge.
There are countless ways to apply Gen AI within your business workflows, products, or services. However, the current hype is creating confusion and pressure, leaving CXOs overwhelmed.
To clear this noise, we've scrutinized leading industry reports to provide a clearer perspective. Trusted by over 30 CIOs, this playbook offers practical steps to help organizations navigate the complex transformation brought about by generative AI. It emphasizes the need to balance innovation with risk, covering valuable use cases, build vs. buy decisions, and responsible scaling.
This guide is divided into two parts for ease of understanding.
- The first part provides an overview of the current Gen AI landscape, including vital research and analysis to support informed decision-making. It also introduces the initial three stages of the 'Gen AI Innovation Lifecycle,' aiding in the setup and execution of Ideation and Scoping workshops.
- The second part delves deeper into the systems necessary for building, iterating, and scaling your Gen AI projects.
As technology evolves rapidly, our understanding of 'what is possible' has been refined through practical experience and learning over the past year. This playbook uses proven frameworks and best practices to help business leaders in setting up pilots and carefully integrating generative AI into the organization's culture.
Executive Summary (TLDR)
[fs-toc-omit] Unveiling Generative AI's Potential
Generative AI's potential spans from creating high-fidelity content to pioneering scientific advancements. This section demystifies the technology, exploring core concepts, market applications, and real-world use cases. It offers insights on strategic AI integration, highlighting significant business impacts and addressing challenges to help executives make informed, impactful decisions.
[fs-toc-omit] Transformative AI in Leading Industries
Learn how leading companies like Moderna, Oscar Health, JP Morgan, and IKEA are leveraging Generative AI to drive innovation and efficiency. Discover practical applications in healthcare, banking, and retail that enhance workflows, customer interactions, and strategic decision-making. Dive into real-world use cases showcasing AI's transformative potential across industries.
[fs-toc-omit] World-Changing Tools* - Disclaimers Everywhere
Generative AI offers immense potential but carries significant risks, from data privacy breaches to security threats. Industry leaders are categorizing these risks and stressing the importance of robust governance. Read on to navigate these challenges and responsibly harness AI's transformative power.
[fs-toc-omit] Introducing the GenAl Skill Spectrum
Navigating Generative AI can be daunting. Our Generative AI Skill Spectrum framework simplifies this by helping organizations align AI initiatives with strategic goals, assess use cases, and manage risks. Learn how this tool aids in decision-making, model comparison, and stakeholder communication, ensuring effective AI integration and innovation. Discover how the Skill Spectrum can transform your AI journey.
[fs-toc-omit] GenAI Innovation Lifecycle
Successfully integrating AI requires a structured approach. Our GenAI Innovation Lifecycle provides a detailed, six-stage framework, from ideation to execution and evaluation. Leveraging Design Thinking and Lean Startup methodologies, this guide helps you launch AI pilots, gain stakeholder confidence, and ensure sustainable AI integration. Explore how to navigate AI opportunities effectively and prepare your enterprise for large-scale adoption.
[fs-toc-omit] Ideate
As the first stage in AI Alignment, ideation is crucial for identifying high-value AI use cases. Move beyond technical POCs by engaging business stakeholders early, ensuring alignment with strategic goals. Conduct cross-functional ideation workshops, leverage the Skill Spectrum, and generate diverse ideas across efficiency, cost, governance, growth, experience, and innovation. Build an 'Idea Pool' to capture and prioritize insights.
[fs-toc-omit] Prioritize
Effective prioritization is crucial to align AI use cases with strategic goals. By evaluating business value and organizational readiness, this stage helps focus on high-impact, feasible projects. Learn how to balance risks and rewards, ensuring your AI initiatives deliver maximum value and drive strategic growth. Dive in to discover the methodology and tools for success.
[fs-toc-omit] Design and Scope
Transitioning from ideation to implementation requires a detailed design and scope. Focus on single, well-structured workflows, define clear success metrics, and create comprehensive opportunity cards. This stage ensures actionable steps for successful AI projects, guiding you to make informed 'Go/No Go' decisions. Learn how to streamline AI integration with practical, targeted sprints.
[fs-toc-omit] What's next - AI Readiness
Unlocking the full potential of GenAI requires strategic AI Readiness. This stage focuses on establishing robust foundations and enhancing organizational maturity for AI initiatives. Learn how to validate operations, upgrade technology stacks, and implement continuous development models. Discover the essential steps to scale AI solutions effectively and manage risks. Dive into part two for comprehensive strategies and best practices.
Overview of the Generative AI Landscape
On the Lex Fridman Podcast, Jeff Bezos described Large Language Models (LLMs) and Gen AI as discoveries rather than inventions, underscoring their expansive applications and, often unpredictable, potential. Their human-like ability to converse, comprehend, and create has captured imaginations, and rightly so. The power of Generative AI extends from creating high-fidelity digital content to pioneering advancements in science and medical research, hinting at a future rich with untapped possibilities. Even if familiar, understanding the technology's core strengths and areas needing controlled augmentation is vital. Armed with this, a leader can make informed decisions, from choosing a use case to scaling AI operations.
{{playbook-lead}}
Where is it being used?
It is important to bear in mind that Gen Al, LLMs, or GPTs, though being used predominantly nowadays, represent just an overlapping segment of the overall Al landscape (represented by the concentric circles below).
McKinsey's study, which analyzed over 60 organizational use cases across 16 business functions, highlighted four areas:
- Customer operations,
- Marketing and sales,
- Software engineering, and
- Research and development.
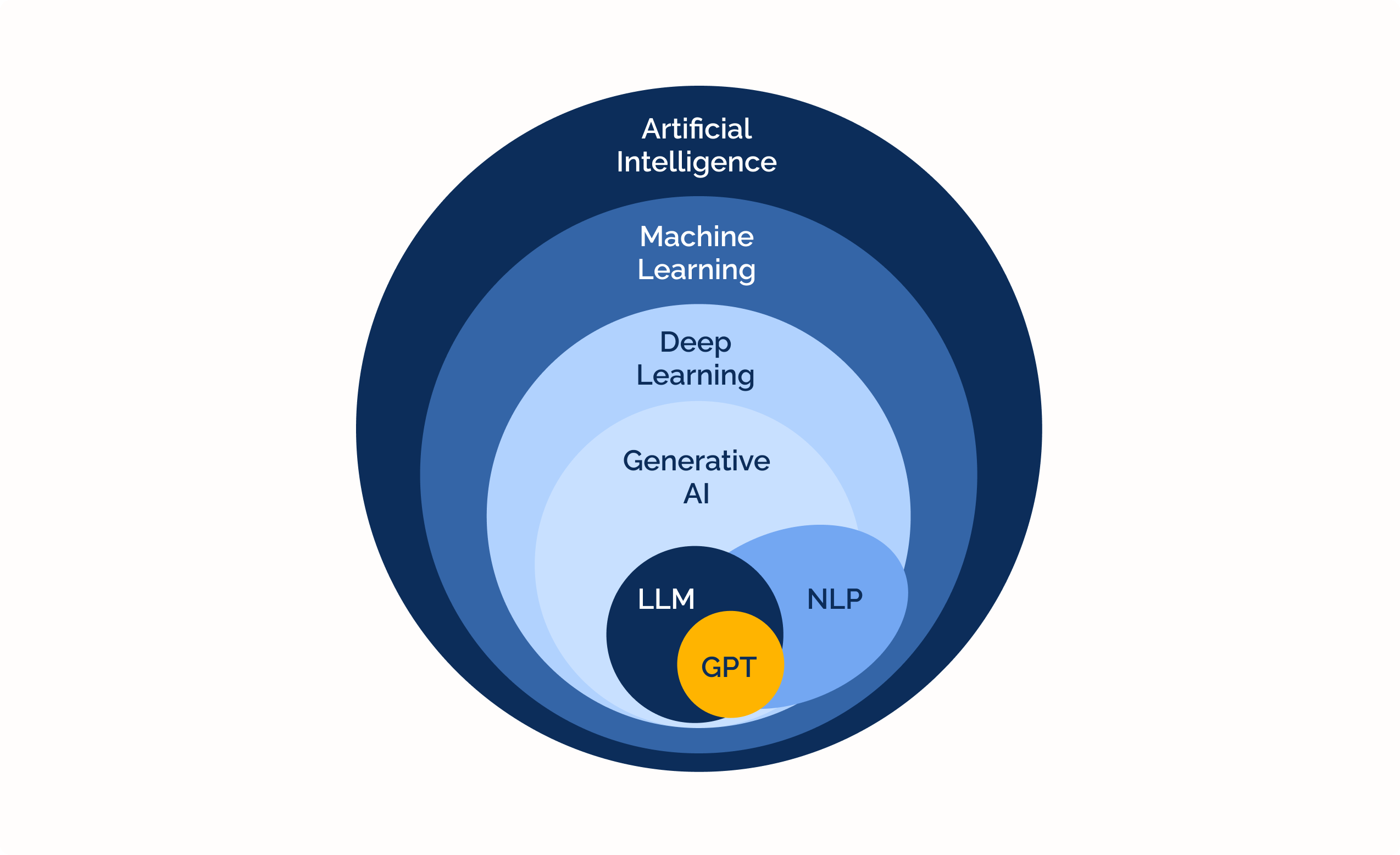
These areas could represent about 75% of the total annual value (estimated to be between $2.6 trillion and $4.4 trillion) derived from Generative AI and would serve as an excellent starting point.
"There is an AI for That", a renowned AI aggregator platform with over 2 million monthly active users, maintains a Job Impact Index. This index tracks novel AI tools being developed and their influence on various job types.
Analyzing the top 10% of AIs developed and published [as illustrated in the figure below] provides insight into popular use case domains.
The findings emphasize the transformative influence of AI on the workplace. A systematic study to understand the impact on various roles and seniority levels within your organization can help strategically navigate the integration of AI technologies while mitigating potential disruptions.
According to Deloitte's key findings, most organizations surveyed are prioritizing tactical benefits like enhancing efficiency/productivity (56%) and reducing costs (35%). Undeniably, boosting productivity and efficiency can drive transformative changes, especially considering the immediate benefits that generative AI can potentially offer.
However, the greatest value and strategic differentiation will likely come from using the technology to innovate business - first by creating new products, services, or capabilities that would otherwise be impossible, and second, by introducing new business models and work methods across an organization

How is it being used?
CIOs globally see information synthesis and discoverability as the most important business applications of Gen AI.
In our work with financial services and healthcare organizations, we have created Q&A bots, data processing utilities, and data augmentation using AI-driven search capabilities.
Further analysis of real-world Gen AI use cases of leading foundational models and industry reports also suggests that the concentration of apps being built are bots, both for customers and employees using existing knowledge management sources.
A significant effort is also being made towards synthetic data generation and fine-tuning models through machine learning applications. The following chart showcases the relevance of generative AI applications across modalities.
While this provides a lens to understand forms of tech adoption, let's check out a few examples of how industry leaders are leveraging it.

Moderna & Oscar set the stage for Gen AI in Healthcare and Life sciences
In 2023, Moderna, a leader in global biotechnology, launched an internal AI chatbot, mChat, powered by OpenAI's API. With over 80% of employees adopting it, mChat paved the way for broader implementation of ChatGPT Enterprise.
This allowed Moderna's staff to develop their own GPTs and create applications across a variety of business processes and teams, thus improving workflows, boosting efficiency, and accelerating innovation
Specific GPTs include:
- Dose ID - A clinical data-analysis assistant for the clinical study team, which enhances the team's clinical judgment and decision-making. Dose ID uses ChatGPT Enterprise to review, analyze, integrate, and visualize large datasets, reducing the time needed for processing and analyzing such data from weeks to days.
- The legal team has fully embraced the tool, using it to develop the Contract Companion GPT for clear, readable contract summaries, and the Policy Bot GPT for quick internal policy queries. This has expedited compliance and allowed the team to concentrate more on high-priority cases.
- Moderna's corporate brand team has also designed its own GPT to prepare slides for quarterly earnings calls and to simplify complex biotech terms for investor communications. This enhances the team's ability to effectively communicate Moderna's story to a variety of audiences.
- Furthermore, Moderna's ambitious product launch plans are supported by their AI-driven approach.
- They aim to bring up to 15 new products to market in the next five years using their mRNA medicine platform, with AI playing a vital role.
Moderna's tech-forward approach, combined with its strategic decision-making, developed a culture of learning and curiosity, thereby driving widespread adoption of AI within the company.
Oscar Health, a healthcare insurance technology company, identified an opportunity to use LLMs to translate the complexities of the real-world healthcare industry into clear digitized plans. Here are some specific ways Oscar is using generative AI:
- Clinical Documentation: Utilizing OpenAI's API, Oscar has cut the time spent medical conversations and reviewing lab tests by nearly 40%, freeing up documentingclinicians to focus on more critical tasks. Research suggests GPT-4 can boost productivity by up to 90% in certain instances.
- Claims Assistant: Due to numerous contractual variables, understanding a claim's life story is complex. Oscar developed an assistant that navigates the claim trace efficiently, automating patient claim inquiries. It has halved the escalation resolution time for the claims processing team, with equal or superior accuracy to humans. Oscar's goal is to automate the investigation of 48,000 tickets annually.
- Medical Record Data: Medical records contain critical patient information but are typically written in highly unstructured and messy natural language. For patients with the most complex and acute health situations, these records can be as long as 500 pages. Finding relevant information in a medical record is like finding a needle in a haystack. With OpenAI’s API, Oscar has developed an assistant and search that can efficiently navigate these records.
JP Morgan leads by example
J.P. Morgan (JPMC or JPMorgan) has recently secured the top position on the Evident AI Index , a public benchmark of major banks' AI maturity. The bank has consistently invested in AI, maintaining a skilled team and modernizing its technology infrastructure in line with AI's growth and needs.
They claim to have developed over 300 AI use cases in areas such as risk management, marketing, user experience, and fraud detection. JPMC has consistently led the way in using technology for large-scale transformation, inspiring the industry.
It is also collaborating with regulators to implement AI safely, sustainably, and ethically across the banking and financial services sector.
Let us look at some of their Initiatives:
- JPMorgan launched IndexGPT with the aim of assisting customers in making informed investment decisions. It will offer access to major investment products in the market, allowing customers to select based on their risk tolerance and financial health. Banks and fintechs can build custom applications using IndexGPT, which is expected to launch between 2026 and 2027.
- JPMorgan also introduced DocLLM, an LLM extension designed for comprehensive document understanding. In an effort to transform the generative pre-training landscape, DocLLM incorporates spatial layout information, going beyond traditional models. This provides an efficient solution for processing visually complex documents.
- The bank has introduced an AI-assisted Cash Flow Intelligence tool.
- This tool aids corporate treasuries in analyzing and forecasting cash flows. It is currently in use by 2,500 clients. Some have reported a 90% reduction in manual work related to categorizing and visualizing payment flows.The tool also enhances processing speed and reduces false positives, thereby speeding up liquidity management decisions.
- Conversational Analytics Assistant: JPMC has piloted a natural language assistant that allows corporate treasures to interact with payments data, making complicated data accessible in real-time. The prototype is trained to understand the context of payments and the corporate treasurer workflow.
- Customized Research: J.P. Morgan's Corporate & Investment Bank utilizes machine learning to personalize the digital experience of its research platform, J.P. Morgan Markets. The platform produces over 10,000 pieces of research a year. Machine learning techniques are used to provide each client with a customized portal offering unique and relevant research.
- Generating synthetic data sets using Gen AI: J.P. Morgan's AI Research team has identified various methods for generating synthetic data sets, tailored to different data types and scenarios. This aids the advancement of AI research in finance, particularly when access to real data is limited in cases such as fraud detection or when more varied data is needed related to risk mitigation.
- COiN, or Contract Intelligence, is designed to interpret commercial loan agreements. The automated system has freed up human employees to focus on more complex and value-added tasks and also minimized mistakes due to human errors. COiN is able to process 12,000 contacts annually and save approximately 360,000 hours of review for the firm's legal teams.
- J.P. Morgan initiated several pilots intending to use Gen AI to enhance customer support. This includes improving 'know your client' (KYC) processes by aiding call center agents in quickly understanding customer conversations and providing timely resolutions. Additionally, they are implementing an AI-driven virtual assistant that empowers users with deeper-level questioning and real-time responses information, ensuring a consistent customer service experience across various channels.
IKEA - AI-led retail innovation
Global furniture retailer, IKEA, has long been a leader in tech-driven retail innovation. What sets IKEA apart is their laser-focused approach, clearly defining their goals before investing in technological advances.
IKEA's innovation doesn't revolve around individual solutions or heavy investment in AI technology simply for the sake of AI. Instead, they focus on a core area of the business, be it front or back end, and thoroughly evaluate how it could be transformed.
Let's explore how IKEA is managing the AI revolution.
- AI Design Assistant: IKEA has launched a chatbot powered by ChatGPT, currently available only in the US. Its goal is to provide each customer with their own AI design assistant. The chatbot can answer queries about products and purchases, and also offer personalized design suggestions. Users can express specific home design needs using GPT, and receive customized recommendations. They can even explore IKEA's extensive product catalog, check availability, and find inspiration from design ideas, home transformation stories, and expert furnishing tips.
- IKEA Kreativ: This is an innovative tool that lets users visualize and design their living spaces in a mixed-reality environment. It combines decades of expertise with the latest developments in spatial computing, machine learning and 3D mixed reality technologies.
- IKEA Place: IKEA is using Gen AI to create the virtual store’s products, furniture and even the store itself. This technology is being used to create virtual worlds that are more realistic and interactive than ever before.
- Design & Launch Collections: In collaboration with research and design lab SPACE10, IKEA trained AI models on IKEA catalogs from the 70’s and 80’s to reimagine IKEA’s design heritage for the future. The idea is to bring vintage designs into the future using generative AI.
- Demand Sensing: IKEA is using ‘traditional’ AI to optimize stock levels and improve customer experiences. The tool, piloted in Norway, interprets up to 200 data sources and considers factors such as shopping preferences, in-store visit volumes, and weather forecasts. It aims to reduce customer frustration caused by inaccurate forecasting and extended waiting periods. The tool has significantly improved IKEA’s inventory forecasts, reducing the correction rate from 8% to 2%.
- Staff Training: While customer queries are increasingly managed by bots, IKEA is heavily investing in reskilling its staff in store or for support so that the entire workforce can effectively use AI to solve problems and drive efficiency. One example is staff online or in store assisting customers as home design assistants.
- Ethical AI Task Force: IKEA has created an ethical AI task force made up of experts from across the entire business to ensure this hugely transformative technology is rolled out responsibly.
Why is it not being used extensively?

Recently, a finance worker mistakenly paid out $25 million after a video call with a deep fake posing as the 'Chief Financial Officer'.. While this could be an isolated incident with malicious intent, CIOs and CISOs have been concerned about the widespread use of Gen AI tools because of the multitude of risks for businesses and individuals, as various incidents have shown.
For instance,
- Air Canada faced legal consequences when a chatbot inaccurately informed customers about bereavement discounts.
- Netflix was accused of using AI-generated imagery in a true crime documentary.
- X’s chatbot Grok, mistakenly accused an NBA player of a vandalism spree due to misinterpreting tweets about a game.
- Google had to postpone platform launches due to the production of factually inaccurate content.
Moreover, ongoing lawsuits against tech giants emphasize the problem of unauthorized use of proprietary data for Large Language Model (LLM) training.
These instances highlight a range of reputational, financial, and societal risks that businesses must be aware of before publicly deploying these tools.
Our executive interviews for this paper confirm that while competitive pressure looms, a ‘lack of confidence or trust’ in the results generated by AI is one of the top risks causing organizations to hesitate in scaling AI adoption or pursuing use cases related to top-line value benefits.
Industry leaders, while advocating for an organization-wide Responsible AI governance framework, have started to categorize GenAI-associated risks into these basic areas :
In the rapidly evolving landscape of this emerging tech, several new risks surface that organizations must vigilantly monitor and mitigate.
Among these are Data Poisoning, a type of adversarial attack in which a malicious party intentionally infuses the training or fine-tuning dataset with corrupted or misleading data samples.
Similarly, there's Prompt Injection, a tactic that manipulates the AI model to generate unexpected outcomes by altering the structure or the intrinsic information within the prompts. Another concern is model degeneration or collapse. This typically happens when AI models lean more towards machine-generated data for learning rather than human-generated data, leading to a significant drop in the quality of output over several iterations.
Over time, the accumulation of errors can drastically distort the model's accuracy. Although it is still early days, companies cannot afford to delay developing policies and practices regarding the use of these technologies.
The crucial first step for organizations implementing gen AI use cases is to identify.
potential risks for each case within key risk categories. This allows for an assessment of risk severity versus the overall value benefit.
To understand the coverage of risks, we recommend IBM’s AI Risk Atlas. We'll discuss this step in detail during the 'Design and Scope' phase of the Innovation Life-Cycle.
Organizations that have identified strong business case(s) but are on the decision stage of scaling must commit to continuous efforts to understand and manage the associated risks. Building trust with stakeholders is crucial and will likely demand a significant investment of time and resources, as well as changes in operational strategies.
This commitment is indispensable for organizations to derive sustainable, transformative benefits from generative AI. Any missteps or failures can undermine the confidence of executives, employees, and customers, leading to a shift towards ultra-safe use cases, which pose limited risks but may not fully exploit the technology's potential.
We recommend running a risk-focused sprint, if not already done. More on this when we cover organizational readiness in part 2 of this paper.
The Gen AI Skill Spectrum
The Inception
At Ideas2IT, we strongly believe that Gen AI can democratize AI usage, making AI agents more readily available to stakeholders across an enterprise's workflows. Although it isn't sentient, it behaves in human-like ways.
As AI becomes more prevalent, choosing and evaluating these agents will need to be rethought - it will become akin to hiring human team members.
Just like humans, the strengths of Gen AI also mirror its weaknesses. Therefore, when selecting these AI agents, it's imperative to understand their capabilities, strengths, and weaknesses for optimal performance and alignment with business goals.
We refer to this understanding as the 'Skill Spectrum'.
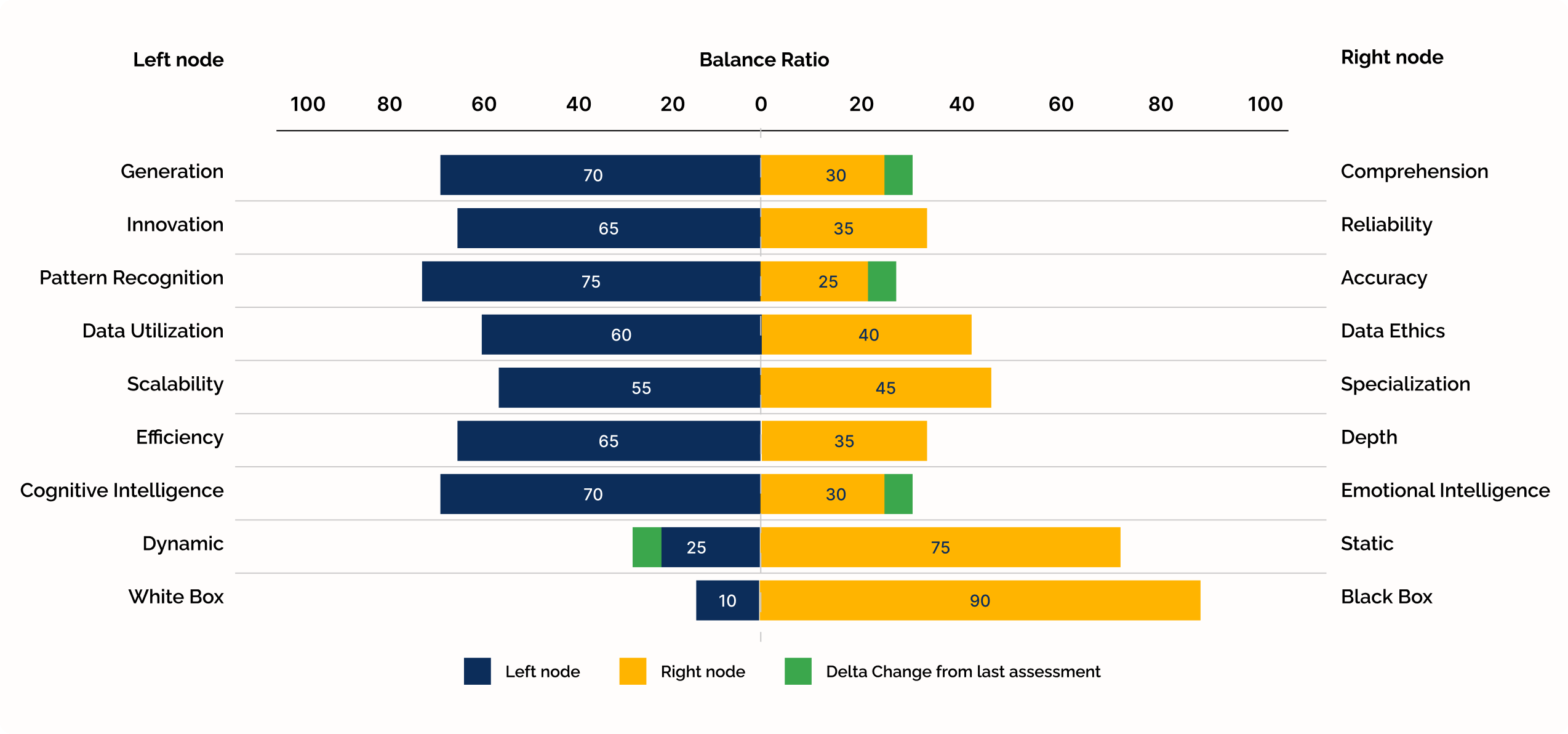
The Framework
The Generative AI Skill Spectrum serves as an insightful framework to gauge the natural capabilities of generative AI models across various dimensions.
This spectrum [pictured above] provides a balanced view of nine opposing traits or skills, each representing contrasting capabilities. It helps organizations identify where an AI model's strengths lie and where it may need further augmentation, development, or oversight.
Left spectrum nodes represent the capabilities towards which the technology is more inclined, while right nodes represent the opposite. Each node symbolizes a capability or trait, rather than strengths or weaknesses. The Balance ratio indicates the technology's natural predisposition.
The above skill spectrum is for OpenAI’s GPT-4. ‘Delta Change’ refers to the shift in the Balance Ratio since the last evaluation compared to GPT-3.5.
The spectrum above identifies both the advantages of the technology and its associated business value, as well as potential challenges and risks that need to be addressed. This is particularly useful when evaluating use cases through the lens of this skill spectrum.
It offers a comprehensive view of the risk vs reward scale and helps understand where the enterprise needs to invest additional effort.
Research Methodology and Maintenance
The Gen AI Skill Spectrum stems from extensive research and systematic in-depth analysis. It’s designed to offer a comprehensive framework for evaluating generative AI capabilities and the solutions built upon them. Apart from benchmark assessments, we consider a mix of subjective reviews and stress tests for each node of the skill spectrum to arrive at the balance ratio.
Let’s consider the Innovation vs. Reliability spectrum where we have determined a balance ratio of 65:35 based on multiple assessments. Our analysts conducted stress tests on GPT-3.5 (Turbo Instruct) and GPT-4 across various tasks for both Innovation and Reliability. They measured results for deviations and error rates, with experts reviewing the findings to finalize ratios and the analysis.
“The models can generate highly creative outputs, often unexpected and original. However, they struggle to maintain consistent quality across repeated requests or over time, yielding erratic or inconsistent outcomes. Both models had difficulty following instructions, but GPT-4 managed to reproduce better results, albeit inconsistently. At the same time, GPT-4 can be guided to produce more consistent and reliable results through effective prompting, similar to how users can achieve more consistent results by specifying a seed value using the "--seed" parameter in their Midjourney prompts.”
As this technology continues to evolve, we stay at the forefront by regularly updating our framework to reflect the latest trends and developments in the field. Our expert team diligently follows the latest research and training methodologies.
We also keep an eye on ground-breaking use cases and innovative applications to emerging risks, recent news, and real-world incidents. We monitor advancements in both open-source and proprietary models, using our expertise to assess each skill area accurately and insightfully.This meticulous process ensures our framework remains relevant and accurately represents the capabilities and limitations of generative AI.
Use of Ideas2IT Skill Spectrum
While the applications of the skill spectrum are being nurtured, the enterprises we applied this with found the following utilities useful.
- Align AI Initiatives with Strategic Objectives: Understanding the inherent strengths and weaknesses of generative AI models can help organizations align their AI ambitions with strategic goals. This ensures effective and appropriate use.
- Use Case Assessment and Recommendations: Develop or evaluate use cases, identifying potential benefits and pitfalls, along with strategies to mitigate them. For example, in a legal document review use case, the risk might involve misinterpretation, the value could lie in faster processing, and the mitigation strategy might involve expert oversight.
- Identifying Gaps and Augmentation Efforts: Determine what it would take to move the balance ratio from the left node to the right or vice versa. For instance, if a company wants to enhance the emotional intelligence of an AI model for customer service, it may need specialized datasets or hybrid systems that involve human supervision.
- Comparing Models, Agents or Solutions: Evaluate and compare two generative AI models for different skills. For instance, when comparing two models for customer engagement use cases, one might excel in "Innovation" while the other performs better in "Specialization or Personalization", making it more suitable.
- Expedite Decision Making: The model assists in decision-making processes throughout the Innovation life-cycle, related to use case selection, solution scoping, and build vs buy decisions, supporting the iterative and agile nature of innovation within enterprises.
- Improving Stakeholder Communication: The framework’s analysis and reports, when used in the scenarios listed above, helped build a common consensus. It served as a valuable communication tool, facilitating clear discussions about AI capabilities and limitations across all stakeholders, from technical teams to executive leadership.
In the following sections, we will explore the Innovation Lifecycle for GenAI and cover how the Skill Spectrum will serve as a key tool in this lifecycle, helping businesses identify and prioritize use cases, align AI initiatives with business value, and manage the risks associated with generative AI to drive sustainable innovation and value.
To learn how the Skill Spectrum framework can help you in this journey and how it can be tailored to your specific needs and applications, we encourage you to connect here.
The Gen AI Innovation Lifecycle
Successfully integrating AI across an enterprise is a complex task. IT leaders need a systematic approach to navigate opportunities and avoid impulsive responses to AI hype. A detailed implementation plan, tailored to the organization's maturity, is essential.
The playbook is designed to guide organizations in launching AI pilots and responsibly expanding usage. Derived from Design Thinking and Lean Startup Methodologies, this framework has proven effective in piloting the technology, gaining stakeholder confidence, and understanding the role of Gen AI in the future of the enterprise.
The Innovation Lifecycle Model encompasses six iterative stages, each with specific tactics, tools and techniques to ensure systematic and sustainable technology integration within your organization.
- Ideate: Generate numerous use case ideas across various business units in your enterprise, emphasizing maximum business value.
- Prioritize: Select a few use cases with high alignment to LLM's natural capabilities that provide maximum business value without significantly increasing risk, and score high on organizational readiness.
- Design & Scope: Define the hypothesis, KPIs, and risks. Design the workflow and scope of the pilot. Reverse engineer the timeline to decide on the next steps.
- Assemble Resources: Form a dedicated team for the pilot, including business partners, developers, front-end designers, AI experts, tools, and models.
- Execute: Build, test, and refine iteratively. Publicize outcomes to foster hands-on acceptance, ensuring the pilot aligns with the set objectives.
- Monitor & Evaluate: Quantify the value derived and continuously assess the fit. Strengthen foundations and decide whether to scale responsibly.
The first three stages, which focus on your organization's AI Alignment (discussed in part 1 of this paper), are designed to be executed through targeted workshops. These workshops aim to map out the business benefits you hope to achieve from AI.
The following three stages (discussed in part 2 of this paper) focus on your organization's AI Readiness. This is crucial for continuous development, risk mitigation, and preparing your enterprise for large-scale adoption.
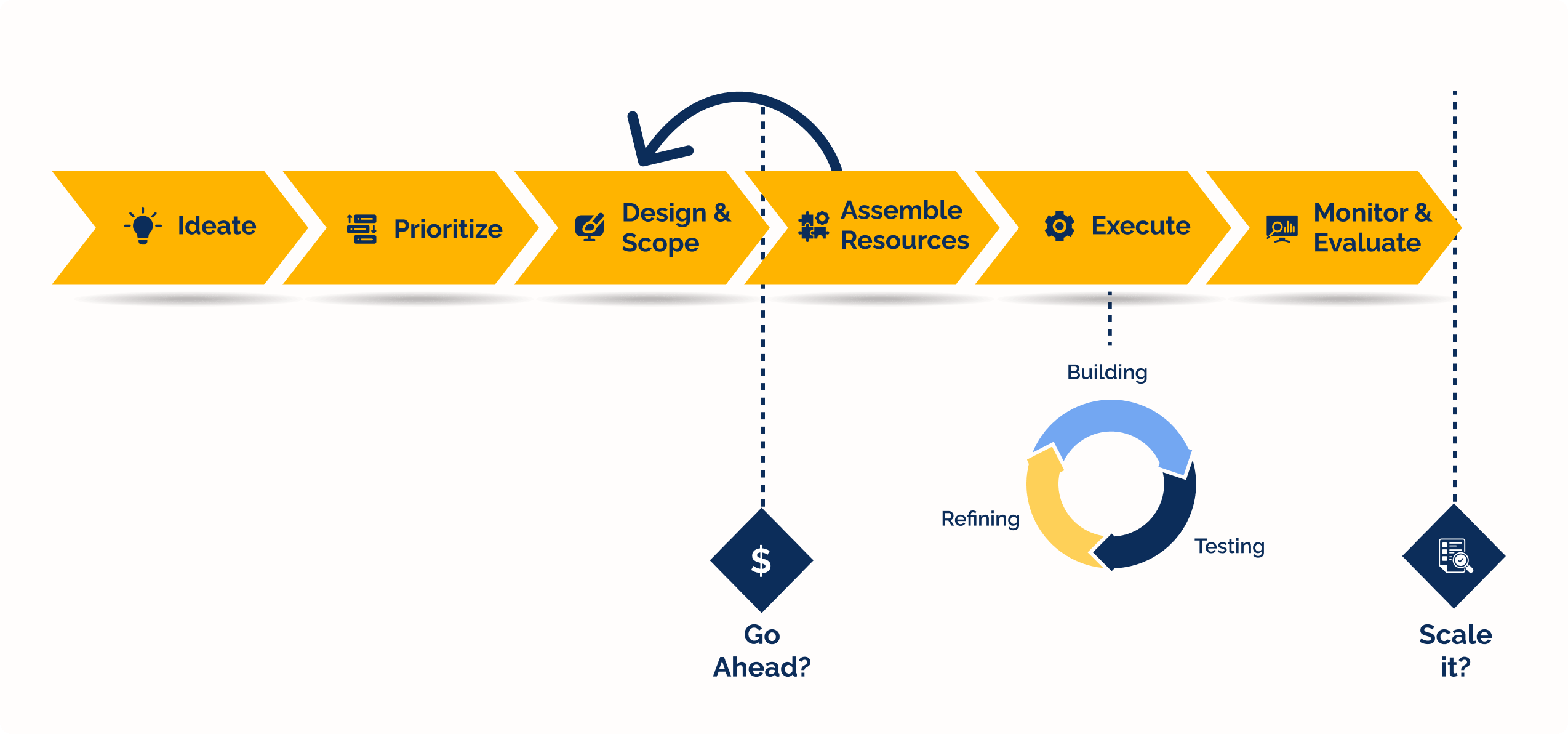
1. Ideate
You have been asked to identify the right use cases to build the pipeline for Generative AI. It’s crucial to note that we have moved past the stage of creating technical proof of concepts (POCs) which only demonstrate that the technology works. These POCs can give a misleading sense of assurance.
To prevent this misconception, it's important to engage the business from the very start of your journey, ensuring that the entire endeavor aligns with business value.
Methodology
A systematic approach aligned with business value will help maximize the benefits of the technology within the organization or the department. We recommend running Ideation workshops with the business.
- Having a core cross-functional working group for Gen AI pilots and the Continuous Innovation lifecycle is imperative. We suggest identifying ‘Executive Sponsors’ and ‘Department Champions’ for Ideation along with AI and Tech Experts. Ideal candidates are those with both interest and influence.
- Schedule workshops and start the sessions to establish a ‘shared understanding’ of the technology. We have found that introducing the Skill Spectrum is a go-to method for enterprises we work with, to call out the natural capabilities of the tech, and highlight its potential value and associated risks.
- Do not restrict the flow of Ideas. Push for use cases beyond incremental improvements to their processes and imagine how the tech could lead to transforming the business. Ask each contributor to consider and capture how the use case positively contributes to one or more strategic objectives of their department or organization, rather than focusing on individual or team performance indicators. Think along the lines of operational excellence and competitor differentiators.
- The value that gen AI use cases can enable could be bucketed in one or more of these six dimensions: Process Efficiency, Cost Reduction, Improved Governance, Growth, Better Stakeholder Experience, and Innovation. Keep a printout of the tech's key modalities handy. [Text, Code, Image, Video, Audio, 3d]
- If you’re stuck, refer to case studies from competition, horizontal or vertical players, or even a completely different industry/workflow for inspiration. Leverage our Use Cases dossier to get started.
- In our workshops, one technique we cover for divergent thinking is asking yourself, "If only I had someone (or a team) to turn ____ into ____, I would ____." Depending on your interpretation of 'I would', this technique can yield immediate efficiency-driven to mid-term strategic use cases.
- Finally, sort and group ideas and prepare an organization-wide running list - An ‘Idea Pool’ which minimally captures the following:
- Title, Enterprise Workflow, Description, Owner, Sponsor, Business Value. A definitive spreadsheet is available to download in our Gen AI Innovation Toolkit.
The AI-First Way
There are specialized brainstorming co-pilots that are being trained on organizational data to help generate ideas, essentially expanding the top-of-the-funnel flow for an idea pipeline.
{{ideation}}
2. Prioritize:
If done well, the Ideation stage would provide a long list of Gen AI use cases from a lens of alignment with business value, inherent technological capabilities, and potential risks. Many enterprises we collaborated with preferred to prioritize use cases on a 2X2 matrix of Impact vs. Effort.
However, we have observed that certain sectors, notably healthcare and financial services, often avoid high-impact-low-effort use cases due to perceived risks and challenges discussed earlier.
To counter this, we advocate for a Business Value vs Organizational Readiness prioritization technique, which ensures no high-impact use case is dismissed prematurely. Let's understand this in detail.
Methodology
A prioritization session should also be conducted with the same working group and ideally, immediately after the Ideation session to ensure a balance of business value, risk and readiness is being assessed as a group.
- Identify 2 to 3 Business value areas (from the dimensions discussed above) the use case impacts positively. For example in the case of the enterprise workflow for Software Engineering, if one chooses to build a ‘Developer Assistant’, the potential business value areas are:
- Process Efficiency - Assist developers in writing, maintaining and deploying code across different environments. Thereby reducing the manual work and time.
- Improved Governance - Developers maintain a consistent experience across platforms by ensuring each deployment is as per quality and guidelines of the specific environment.
- Improved Governance - Developers maintain a consistent experience across platforms by ensuring each deployment is as per quality and guidelines of the specific environment.
- Capture the Business Value Areas and update the ‘Idea Pool’ worksheet in the Innovation toolkit. Arrange the use cases as per the Business Value Impact (Low, Medium, High) on the 3X2 grid for Value vs. Readiness.
- After this, proceed to the 'Readiness Assessment'. The organization's AI Readiness requires the working group to evaluate the organization's risk management capabilities, along with identifying technical or resource feasibility across four areas: (a) Data, (b) People, (c) Tech and Infrastructure, and (d) processes. Separating 'Risk' from the overall technical feasibility allows for viewing the use cases on a Risk vs. Reward scale as well.
- During the discussion, rearrange individual or clustered use cases/ideas basis common consensus of the working group participating in the workshop. Debate the readiness in all four areas for both risk management and technical feasibility.
- A guide to scoring your use cases on business value and organizational readiness is available as part of the toolkit. The set of questions in the toolkit is for reference only and as a starting point to build consensus.
- Continuing on the use case examples for the enterprise workflow - software engineering. The figure below illustrates what a prioritization might look like. Please note that the size of the bubble here is the ‘Skill Spectrum’ score.
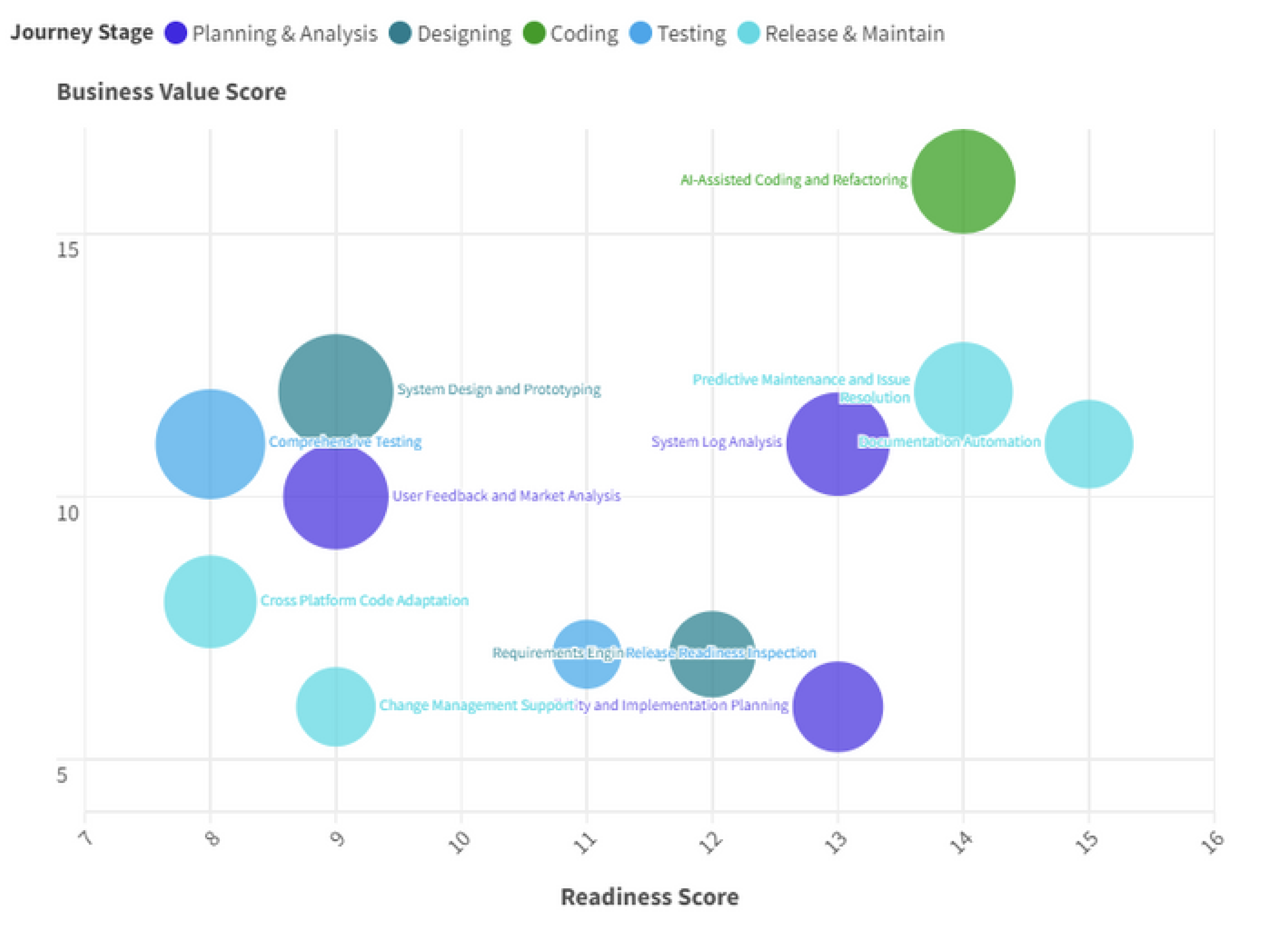
The AI-First Way
Use the generic intelligence capability of LLMs and build a set of custom prompts or an Agent/GPT to take a user's use case input, score each issue/opportunity, and generate an Opportunity Card.
{{priortize}}
3. Design and Scope
As we move into the Design and Scoping work for a portfolio of prioritized use case(s), it’s crucial to transition into practical, actionable steps that ensure a successful AI Implementation. This stage is about scoping a tightly defined sprint that can deliver measurable results and provide valuable insights for broader AI deployment.
Methodology
For enterprises initiating their innovation journey, we recommend a swift design sprint. This approach enables teams to craft opportunity cards for each use case, maintaining an optimal project scope. Here are our distilled insights:
- Focus on a Single Workflow: Resist the temptation to automate complex, cross-departmental workflows. Concentrate on one self-contained workflow within a specific team's domain. This approach curtails uncertainties and risks, streamlines data requirements, training, and change management, and establishes clear, user-group-specific success metrics.
- Choose a Well-Structured and Mature Process: Opt for a well-documented and quantifiable business process. The ideal process will have clear inputs, outputs, and steps, along with established performance metrics. It should be managed by a team with a thorough understanding of the existing operations and challenges.
- Keep the Scope Tight: Ensure the pilot project remains tightly contained. Aim for a one to two-week sprint for scope completion. Starting within a controlled environment minimizes disruption and provides concentrated insights, guiding an incremental expansion strategy.
For enterprises with established operations and governance, the opportunity card can be broadened to encompass detailed user stories within a contained or cross-functional workflow.
The Opportunity Card, a key element of the AI project or sprint, encapsulates essential components and offers a shared overview for decision-making. The critical elements of an Opportunity Card are:
- Title & Description: Provide a succinct 'Title,' 'Description,' and an identification of the ‘issue/opportunity,' following an in-depth review of the use case.
- Business Value & Success Metrics: Illuminate the business value areas identified in earlier stages. Establish a consensus on the project's alignment with the broader enterprise goals and create the value hypothesis. This is a projection on the AI use case's impact on specific business KPIs or Success Metrics. For example, measurable KPIs for 'Process Efficiency' and 'Improved Governance' areas could encompass:
- Decreased code maintenance time
- Enhanced developer output
- Improved AI-generated code accuracy
- Diminished deployment error rates
- Risk & Mitigation: The core team should pinpoint the risks tied to the use case and implement mitigations and controls. By distinguishing risks from the prioritization stage, risk coverage receives concentrated effort, even if minimal. Broad risk categories should be discussed, and enterprises may find categorizing risks into 'Evolving,' 'Internal,' and 'Systematic' mitigation buckets beneficial. We will delve into this in the 'Monitor and Evaluate' stage of the innovation lifecycle.
- Data and Technical Feasibility: Begin by outlining the data required for your use case. Assess the quality and availability of the necessary data for your AI model training and inference. Establish robust data handling through data pipelines, storage solutions, and integration capabilities. Define the selection criteria for AI models and lay out your training processes. Consider the cost-effectiveness and risk factors of leveraging pre-trained models versus building from scratch. Identify the requisite technology and tools, development environments, and integration points. Ensure that all technical aspects are covered by creating a comprehensive checklist, leveraging the expertise of data scientists and engineers.
- For instance, in our 'Assistant Developer' use case, the data requirements could include access to existing codebases, deployment scripts, and platform-specific guidelines. The overall technology stack could consist of IDE integration like Visual Studio Code, AI frameworks like GPT-4, and deployment tools such as Jenkins or Kubernetes.
- Resource and Project Planning: Maintain a consistent core working group, involving other parts of the organization at different lifecycle stages. The organization's Innovation and AI Readiness significantly influence the feasibility and resource planning, which in turn impacts the scope of work. Therefore, these two stages are usually interchangeable and iterative until an enterprise establishes a stable process to define them. More on this in the 'Assemble Resources' stage of the Innovation Lifecycle.
- Plan for a smooth integration of AI solutions into existing business processes. Map out the scope, milestones, timelines, and responsibilities. We recommend using Agile and Scrum frameworks for general AI initiatives. These are sprint plans for enterprises with mature operations.
- Stakeholder and Change Management: Establish governance committees to oversee the AI project, ensuring alignment with business goals and adherence to standards.
- Draft a comprehensive communication plan to keep stakeholders informed and engaged throughout the project lifecycle.

‘Design and Scope’ is a crucial stage in the overall Innovation Lifecycle. The outcome of this stage should allow you to make an informed ‘Go/No Go’ decision for the pilot, project, or set of user stories within a sprint. Those hoping to skip some of this necessary work while launching Gen AI projects are likely to meet disappointing results, or worse, may end up creating some serious damage due to the known risks and challenges associated with the tech.
The AI-First Way
Use GenAI's capabilities to extrapolate high-level concepts into detailed epics, user stories, and acceptance criteria. GenAI's text-to-code and thus design capabilities also allow for the rapid creation and iteration of wireframes or even low-fidelity prototypes based on specified parameters.
{{design}}
AI Readiness
The potential of GenAI excites many strategic decision-makers, with CIOs and CTOs playing a crucial role in realizing this value. However, we have observed that many enterprises hesitate at the decision stage of scaling adoption. Despite the value of pilot use cases, they're hesitant due to risks and challenges associated with organization-wide or customer-facing workflows.
Before deciding on large-scale adoption, enterprises often want to validate operations and secure approvals from various departments. Once they gain confidence, they can then scale in many cases.
The Gen AI puzzle has many pieces that need consideration before widespread adoption. Therefore, we divided the stages of the GenAI
Innovation Lifecycle into two interconnected yet distinct buckets: AI Alignment and AI Readiness.
Having completed the first part of this guide on AI Alignment, typically undertaken during focused workshops, we now delve into the equally vital AI Readiness. Unlike part one, part two is about continuous efforts to establish robust foundations, enhancing your organization's maturity to manage Gen AI initiatives.
These stages are not isolated. Depending on your organization's maturity, you may find yourself alternating between alignment and readiness phases. This adaptability enables the scaling of complex use cases and the seamless integration of advanced AI capabilities.
.png)
{{stingray}}
In our ongoing dialogue with industry leaders and our comprehensive analysis of generative AI initiatives across various organizations, we have identified key steps leaders can adopt to effectively orchestrate technology and data, scale solutions, and manage risk.In the second part of this series, we delve into the following aspects:
- We pose targeted questions to gauge your position on the AI Readiness scale and explore the implications of becoming an AI-Native organization.
- We discuss the formation of a centralized, cross-functional Gen AI platform team. This team would be responsible for approving vendors, providing expertise, and approving (whether built or bought) models for on-demand product and application teams.
- We recommend upgrading your technology stack to integrate and manage gen AI models successfully. This would facilitate their orchestration with existing AI/ML models, applications, and data sources.
- We advise fine-tuning your data architecture to ensure access to high-quality data by processing both structured and unstructured data sources.
- We suggest reimagining the technology function by incorporating generative AI capabilities into the software development life cycle for iterative execution. This would reduce technical debt and manual effort in IT operations. Additionally, we provide an overview of LLMOps from industry leaders and discuss the evolving role of Quality Assurance (QA) teams in the GenAI landscape.
- We emphasize the need for robust governance to monitor performance, gather evidence for scaling or discontinuing initiatives, evaluate the emerging risk landscape, and establish persistent mitigation practices to build trust in the ecosystem.
- As organizations gain a deeper understanding of the strengths and potential use cases of Generative AI, they also recognize the underlying prerequisites within their organization to fully harness the transformative power of Gen AI. Join us as we explore the journey towards achieving this essential readiness.
References
- https://www.statista.com/outlook/tmo/artificial-intelligence/generative-ai/worldwide
- https://www.youtube.com/watch?v=DcWqzZ3I2cY
- https://www.mckinsey.com/capabilities/mckinsey-digital/our-insights/the-economic-potential-of-generative-ai-the-next-productivity-frontier#business-value
- https://theresanaiforthat.com/job-impact-index/
- https://www2.deloitte.com/content/dam/Deloitte/us/Documents/consulting/us-state-of-gen-ai-report.pdf
- https://cloud.google.com/transform/101-real-world-generative-ai-use-cases-from-industry-leaders
- https://openai.com/index/moderna
- https://openai.com/customer-stories/oscar
- https://evidentinsights.com/ai-index/
- https://www.jpmorgan.com/insights/technology/artificial-intelligence/championing-the-industrial-revolution
- https://www.bloomberg.com/news/articles/2023-11-09/jpmorgan-is-working-with-us-regulators-on-generative-ai-pilot-projects
- https://www.bloomberg.com/news/articles/2024-05-03/jpmorgan-unveils-indexgpt-in-next-wall-street-bid-to-tap-ai-boom
- https://www.jpmorgan.com/technology/artificial-intelligence/initiatives/datasets
- https://www.bloomberg.com/news/articles/2024-03-04/jpmorgan-s-ai-aided-cashflow-model-can-cut-manual-work-by-90
- https://www.jpmorgan.com/insights/payments/analytics-and-insights/genai-virtual-analytics-assistant-treasury
- https://www.jpmorganchase.com/newsroom/stories/tech-investment-could-disrupt-banking
- https://www.jpmorganchase.com/newsroom/stories/tech-investment-could-disrupt-banking
- https://www.jpmorgan.com/technology/technology-blog/synthetic-data-for-real-insights
- https://futurism.com/an-ai-completed-360000-hours-of-finance-work-in-just-seconds
- https://www.ingka.com/newsroom/ikea-launches-new-ai-powered-assistant-in-openai-gpt-store/
- https://www.ikea.com/global/en/stories/design/to-nytillverkad-and-beyond-ikea-space10-and-designers-of-tomorrow-explore-future-with-ai-230420/
- https://edition.cnn.com/2024/02/04/asia/deepfake-cfo-scam-hong-kong-intl-hnk/index.html
- https://tech.co/news/list-ai-failures-mistakes-errors
- https://www.nist.gov/itl/ai-risk-management-framework
- https://www.nist.gov/itl/ai-risk-managementhttps://www.mckinsey.com/capabilities/risk-and-resilience/our-insights/implementing-generative-ai-with-speed-and-safety-framework
- https://dataplatform.cloud.ibm.com/docs/content/wsj/ai-risk-atlas/ai-risk-atlas.html?context=wx&audience=wdp
- https://www.boardofinnovation.com/blog/the-ai-powered-stingray-model-innovation/
[fs-toc-omit] removefromhere
Ideation Checklist:
- Capitalize on the overall enthusiasm and fascination around the technology to conduct ideation workshops to generate use case ideas.
- Form a cross-functional working group, including 'Executive Sponsors' and 'Department Champions'.
- Use the Skill Spectrum to establish a shared understanding of the technology.
- Encourage expansive thinking for use cases, linking to the organization's strategic objectives.
- Create a running list of all ideas - the 'Idea Pool'.
Prioritization Checklist:
- Identify and articulate the specific business value each use case delivers, linking directly to strategic objectives.
- Evaluate readiness across key areas—Data, People, Technology, and Processes. Use a Business Value vs. Organizational Readiness matrix to visually categorize and prioritize use cases.
- Conduct thorough risk assessments for each use case, considering both potential challenges and rewards, to inform better decision-making.
- Use the Skill Spectrum for GenAI alignment, focusing on use cases, for instance, that don't require sharing sensitive information or perfect accuracy.
- In early experimentation, build a portfolio with both feasible and valuable use cases for risk-reward balance. Tackle a small part of a big problem, showing strategic potential.
Design and Scoping Checklist:
- Use the INPUT → PROCESS → OUTPUT model to identify and mitigate risks, determine data and resource needs, and define a pragmatic tech stack.
- Maintain a concise and clear scope to understand the tasks for the sprint/project. Adopt a 'Time Backwards' approach. This helps you plan according to timelines, ensuring controlled iterative development.
- Use externally available foundation models and applications unless specific risks or the need for scalability dictate building or customizing your own model.
- Utilize the 'Opportunity Card' for effective communication and consensus-building.
- Always establish KPIs and benchmarks in line with the test created for the hypothesized business value.
- A Skill Spectrum analysis can identify gaps between your chosen AI model and use case requirements, assisting you in drafting training and augmentation or oversight needs.
The Stingray Model
For enterprises with a matured state of AI readiness, industry leaders recommend adopting or transitioning into Continuous Integration and Continuous Development models of innovation. One such model, known as the "Stingray Model” , is advocated by the Board of Innovation. They suggest that the 'Double Diamond' approach may be outdated, as it heavily relies on human intuition, leading to biases and inefficiencies. Conversely, the "Stingray Model” integrates AI into the innovation process to set deliberate goals, explore a broad range of solutions simultaneously, and iteratively validate practical solutions, thereby increasing efficiency and scalability.