In the rapidly advancing field of Generative AI (Gen AI), maintaining a competitive edge requires more than just keeping up—it demands proactive and strategic measures. As Shantanu Paknikar, Chief Strategy Officer at Ideas2IT and moderator of the session aptly introduced, the journey through the Gen AI hype cycle is nothing short of breathtaking.
With advancements coming at a breakneck speed, it's crucial for organizations to establish robust enterprise guardrails to ensure that progress doesn't lead them astray.
Our Gen AI Blueprint initiative’s first webinar, "Enterprise Guardrails For Successful Generative AI Strategy & Adoption," aimed to equip participants with essential insights on implementing these guardrails effectively. As the velocity of innovation increases, so too must the strength of our guiding principles to avoid potential pitfalls.
We were joined by a distinguished panel of experts:
- Kelly Batlle, Head of Technology at Medtronic Labs,
- Canice Wu, RVP - Portfolio Leader for Financial Services at Salesforce,
- Dr. Arash Kia, Director of Clinical Data Science at Mount Sinai Health System.
This blog provides a recap of the key points discussed during the webinar. Our speakers delved into critical aspects of Gen AI, including data management, governance, and the ethical considerations necessary for successful implementation.
As you explore this summary, you will be equipped with actionable strategies to ensure your Gen AI initiatives are both innovative and secure.
With that said, here’s a quick rundown of the webinar.
Data Management and Governance
In the realm of Generative AI (Gen AI), effective data management and governance are crucial for ensuring both operational excellence and compliance. As Shantanu noted, "Data is the fuel for AI, and guardrails need to start with best practices for data safety."
Dr. Arash Kia kicked things off by emphasizing a transformative approach to data management by rejecting the idea of traditional guardrails and advocating for a real-time sensing system.
As he put it, "Instead of traditional guardrails, we need a sensing system that actively monitors operational conditions and flags irregularities in real-time." This proactive approach allows for continuous assessment and rapid response to issues, essential for maintaining the integrity of AI systems.
He also highlighted the importance of addressing data quality variations in healthcare, noting, "Different practitioners have different ways of evaluating patients, which can significantly impact the performance of Gen AI systems."
Kelly Batlle underscored the critical role of data integrity and regulatory compliance in managing Gen AI projects. At Medtronic Labs, their Spice platform plays a central role in ensuring data quality through stringent validation protocols and monitoring practices.
Kelly emphasized, "We have validation protocols built within the application, ensuring accuracy and completeness of the data set." Her approach includes adhering to international standards like GDPR and HIPAA, which she described as essential not just for compliance, but for building user trust.
"Adherence to GDPR and HIPAA standards is crucial for building trust with users by ensuring their data is handled with the utmost care," she explained.
Canice Wu went into managing data privacy and security within the financial sector by highlighting Salesforce's commitment to trust and privacy, stating, "At Salesforce, our core values are very central to what we do, and trust is our number one core value."
Canice discussed the importance of maintaining confidentiality through strategies like data masking and secure handling of private data sources. He noted, "Leveraging public foundational models is beneficial, but keeping private data sources secure is critical to maintaining trust and compliance."
This approach ensures that sensitive information remains protected, even when using third-party or public models.
Ethics, Bias and Fairness in AI
The discussion on ethics, bias, and fairness in AI is critical as it directly impacts the reliability and trustworthiness of AI systems.
Dr. Arash highlighted that bias can originate at both the product and workflow levels. Dr. Kia shared, "One important piece after product design is feature engineering. For clinical effectiveness optimization, we focus on the clinical profile instead of using administrative data or data that can be proxies for socioeconomic status, race, or ethnicity."
This approach helps in reducing biases that might be introduced through indirect variables. He also emphasized the importance of continuous monitoring: "Bias is something that needs to be monitored on an ongoing basis. MLOps is very important here," he explained, pointing out that changes in patient demographics and workflows require constant vigilance to ensure fairness.
Kelly provided a comprehensive view of how ethics and bias are managed in health tech platforms. She stressed a patient-centric approach, stating, "We make sure that the patient is at the center of any decisions we make from a product perspective." This includes ensuring informed consent and data privacy controls are in place before implementing any models.
She also highlighted transparency as a cornerstone of their approach, noting, "We ask, 'Can we actually explain what’s happening here?' with each project." She emphasized that identifying and addressing biases in datasets before modeling is crucial for maintaining public trust.
Furthermore, she mentioned ongoing efforts to develop dedicated governance models for AI ethics as a commitment to responsible AI usage.
Transparency and Accountability
In the realm of AI, transparency and accountability are essential for fostering trust and ensuring the responsible use of technology. Canice provided valuable insights into how these principles are applied, particularly in the context of financial services.
He emphasized that "transparency and accountability are crucial, especially as we start using AI in our relationships with customers and within companies." He highlighted the concept of "trust and verify," which involves having a "human in the loop" to validate and understand AI decisions.
This approach is to ensure that recommendations and decisions made by AI systems can be traced back to their sources, thus reinforcing accountability.
Looking ahead, Canice stressed the importance of maintaining transparency as automated solutions become more prevalent. He noted, "Having that transparency helps build trust," especially when customers seek to understand the rationale behind AI-driven decisions.
This is crucial in scenarios where decisions like loan approvals might impact individuals' lives, and understanding the reasoning behind these decisions is vital for fairness and trust.
As AI continues to evolve, ensuring that users can understand and verify AI-generated recommendations will remain a key focus for enterprises aiming to implement these technologies responsibly.
Gen AI Safety and Regulatory Compliance
A well-structured product development lifecycle, especially in high-stakes fields like healthcare is of utmost importance as emphasized by Dr. Arash. He advocated for a strategic approach that spans from ideation to scaling.
Dr. Arash noted, "We need to think about how we envision the entire lifecycle—from ideation and development to pilot testing and scaling up." He stressed that a robust automated platform for measuring performance and meeting key performance indicators (KPIs) is essential.
Additionally, he highlighted the need for regular feedback loops between stakeholders to ensure both rapid development and effective operationalization of AI products in clinical settings.
Kelly, on managing the proliferation of AI tools within a regulated environment, highlighted the importance of starting with use cases that do not involve sensitive patient data to maintain privacy and regulatory compliance.
Kelly shared, "We focus on doing our organizational due diligence, ensuring we have the right consent, data-sharing terms, and prioritize data privacy and security." She discussed their approach of using publicly available materials for initial projects, which allows them to familiarize themselves with new technologies while adhering to regulatory requirements.
Her team continuously assesses their framework for evaluating Gen AI use cases, focusing on data, technical capabilities, and operational aspects to ensure scalability and sustainability.
On how enterprises can effectively leverage innovation from startups while maintaining safety and compliance, Canice suggested viewing AI solutions as a combination of core applications, models, and data.
He advised, "Understand where your strengths lie and where you might need to bring in external solutions. It’s crucial to base decisions on practical business value and the specific changes you want to achieve."
He noted that startups often focus on specific areas, so aligning their solutions with your enterprise’s needs while maintaining strong governance is key to leveraging their innovations effectively.
Canice further elaborated, "In this journey, remember the classic framework of people, process, and technology, but also add data and governance into the mix. Governance ensures that data is managed securely and that your models and applications are well-regulated. Bringing it all together effectively is key to leveraging innovations from startups while maintaining safety and compliance."
Q&A Session
Once the main session concluded, we transitioned into an engaging Q&A segment. Our panelists addressed pressing audience questions, delving into topics such as governance for AI models, managing skill gaps, and navigating the shift from proof of concept to production in Generative AI.
What additional governance should enterprises implement when leveraging models like GPT-3.5 or GPT-4 for Gen AI solutions?
Dr. Arash: Beyond generic validation (e.g., hallucination rates), implement a business risk management platform. Evaluate the adaptability of the AI tools and ensure they align with existing operational models. Regular benchmarking and a robust framework are essential.
Canice: Governance should balance technical, ethical, and financial aspects. Assess business value to prioritize efforts and address ethical concerns. The governance structure needs to be comprehensive, integrating these facets effectively.
How can enterprises address skill gaps and leverage partner networks in their Generative AI journeys?
Check out our proprietary skill spectrum developed as part of our Gen AI playbook here.
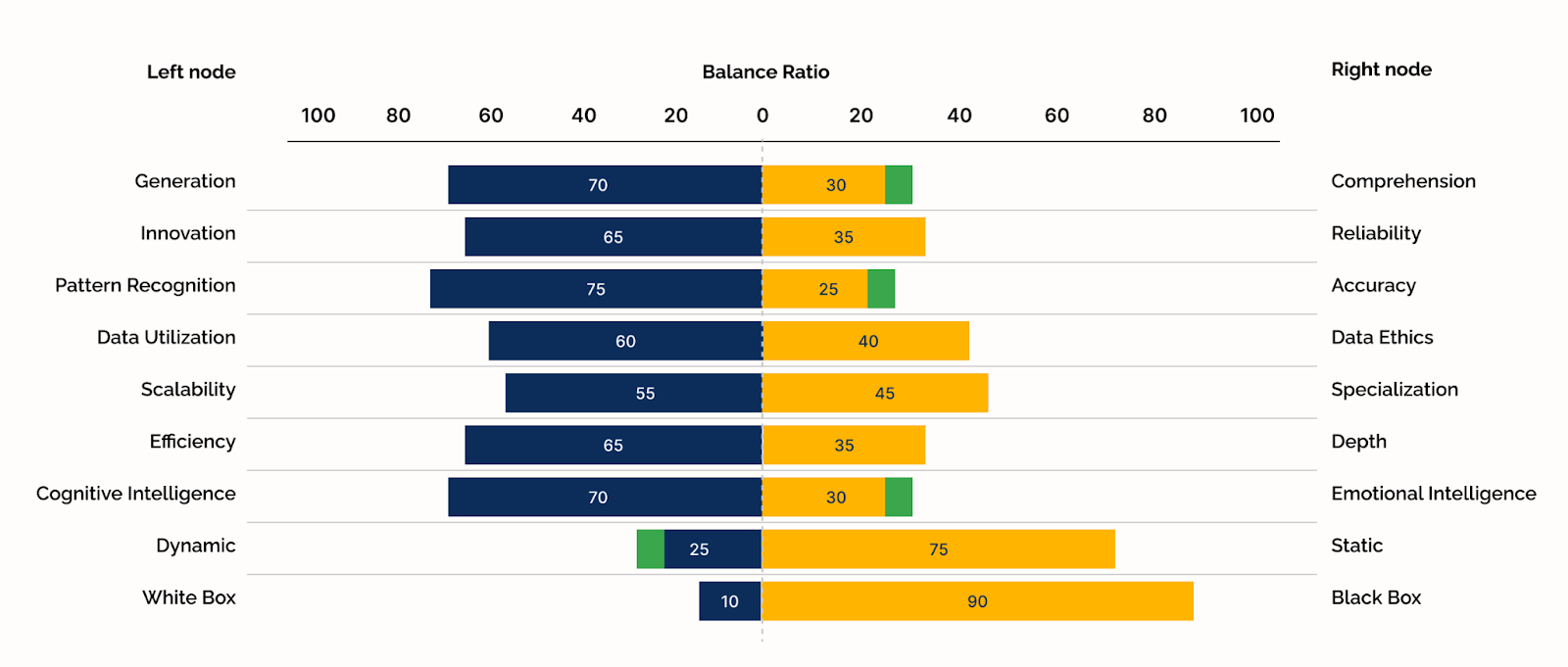
Kelly: Address skill gaps through internal training and curated courses. Partnerships, like those with Ideas2IT, enhance capabilities by providing access to broader expertise and experiences.
What are key considerations for transitioning from a Generative AI proof of concept to a production application?
Arash: Focus on rapid experimentation, pilot testing, and real-time performance evaluation. Implement robust monitoring, ML Ops, and set clear performance metrics. Rigorous testing is crucial for a successful transition.
What margin of error is acceptable in Generative AI, given its unique challenges?
Dr. Arash: Acceptance criteria depend on usability and workflow fit. Balance sensitivity and specificity, and consider class imbalances. Establish criteria with input from clinicians and operational teams, factoring in the cost of false positives.
How should we build a business case for Gen AI given its novelty and the need for budget justification?
Canice: Align the business case with strategic initiatives. Define capabilities, KPIs, and metrics reflecting strategic goals. Use a phased approach—'crawl, walk, run'—to set achievable milestones and adapt as AI evolves. Focus on continuous improvement and innovation.
Elevate Your Generative AI Journey with Ideas2IT
As we conclude today's discussion on the key aspects of Generative AI, Kelly shared her final thoughts on the importance of advancing with both speed and governance. Her advice: prioritize your use cases, experiment in sandbox environments, and embrace continuous learning to drive innovation.
Experimentation, even with use cases that may not move forward, is an integral part of learning and progress.
A heartfelt thank you to our esteemed speakers: Dr. Arash Kia for his insights on AI safety and operationalization, Kelly Batlle for her focus on ethical considerations and data privacy, and Canice Wu for his perspectives on transparency and accountability in financial services. Your expertise and contributions have been invaluable.
Be sure to keep an eye on our LinkedIn for updates on our next webinar in the Gen AI Blueprint series, where we will explore practical use cases of Generative AI - a topic many of our customers are eager to explore.
And while we’re here, don’t miss out on your free copy of The CXO's Playbook for Gen AI - Part 1!