The insurance industry stands on the cusp of a transformative revolution, one powered by the innovative capabilities of generative Artificial Intelligence (AI).
The integration of AI and ML is rapidly transforming industries, reshaping industries, driving operational efficiencies and unlocking new avenues for innovation and growth.
In the insurance sector, generative AI is set to revolutionize traditional practices. The market is expected to grow from USD 346.3 million in 2022 to USD 5,543.1 million by 2032, with a CAGR of 32.9%, according to Market.Biz.
Significance of Generative AI in the Insurance Industry
Why does this matter to the insurance industry? The answer lies in the industry's relentless pursuit of enhanced efficiency, accuracy, and customer-centricity.
Generative AI promises to be the driving force behind these aspirations, offering a myriad of opportunities:
Enhanced Risk Assessment
Generative AI can sift through vast datasets, identifying hidden patterns and risk factors that human underwriters might miss. This translates into more precise risk assessment, reduced fraud, and optimized pricing strategies.
Streamlined Claims Processing
Manual claim processing can be a time-consuming and error-prone endeavor. Generative AI automates this process, leading to quicker claim settlements, improved customer satisfaction, and ultimately, more sales through enhanced trust.
Personalized Customer Experience
Today's insurance customers expect tailored solutions that cater to their unique needs. Generative AI enables insurers to customize policies, recommend coverage options, and deliver personalized experiences that resonate with individual clients.
Generative Artificial Intelligence (AI) stands out as a powerful force poised to redefine the way insurers operate. In this section, we will delve into the fundamental concepts of Generative AI and its applications within the insurance landscape.
How Generative AI Stands Apart from Traditional AI
Creativity and Autonomy:
While traditional AI systems follow predefined rules and rely on labeled data for learning, generative AI has the ability to create entirely new content without explicit programming. It doesn't merely regurgitate existing information; it generates novel outputs.
Unstructured Data Handling:
Traditional AI excels at structured data analysis, whereas generative AI can handle unstructured data types like text and images more effectively. This versatility makes it particularly valuable in contexts where data is diverse and dynamic.
Natural Language Processing (NLP):
Generative AI is exceptionally proficient in natural language generation, allowing it to produce human-like text. This capability has far-reaching implications for customer interactions, content generation, and more.
Top Use Cases for Generative AI in the Insurance
Listed below are the 5 top use cases of Gen AI in insurance:
Claims processing and fraud detection
Generative AI revolutionizes insurance claims processing by automating document assessment and data extraction. This reduces manual effort and processing time, ensuring quicker and more accurate claim settlements.
For example, in property insurance, AI automates assessments for claims arising from natural disasters, streamlining the process for affected policyholders.
Generative AI also detects anomalies and potential fraud by analyzing claims data, summarizing histories, and flagging inconsistencies. This technology enhances efficiency across all claim types, supporting fraud prevention and optimizing workflow through automated data entry and analysis.
Automated underwriting
Generative AI enhances insurance underwriting by automating the analysis of extensive paperwork and customer data. It uses historical documents to identify patterns and trends, enabling personalized policy generation and tailored pricing recommendations. This streamlines the underwriting process, improves accuracy, and lowers overall claims costs.
By analyzing vast datasets, including historical claims and customer profiles, Generative AI assists underwriters in assessing risks and recommending appropriate coverage levels swiftly and accurately. This efficiency both speeds up application processing and enhances customer satisfaction by reducing errors in policy decisions.
Virtual assistants and customer support
Generative AI-powered virtual assistants and chatbots are revolutionizing customer support in the insurance industry. These AI systems provide real-time assistance for policy inquiries, claims updates, and general insurance questions, reducing the workload on human agents and ensuring 24/7 availability.
For instance, virtual assistants on insurance websites guide customers through claims processes and provide essential information instantly.
Moreover, AI-driven chatbots offer immediate responses to policy-related queries, enhance engagement by sending personalized reminders and updates, and contribute to higher customer satisfaction and retention rates.
Personalized products, services, and policies
Generative AI is now personalizing customer interactions and automating tasks traditionally handled by humans. For instance, insurance companies utilize Conversational AI to answer customer inquiries about policy details and coverage options, reducing reliance on live support agents and improving cost efficiency.
By analyzing customer preferences and claims history, Generative AI also recommends tailored insurance products swiftly, enhancing customer satisfaction and accelerating decision-making processes.
Additionally, AI automates the generation of policy documents and provides real-time support through virtual assistants, ensuring prompt service delivery and enhancing overall customer experience.
Cyber insurance and regulatory compliance
Generative AI models are instrumental in enhancing risk management strategies, particularly in cybersecurity and regulatory compliance for insurers. These models simulate cyber threats, helping insurers understand and assess complex risks.
By tailoring cybersecurity insurance policies to individual client needs, generative AI ensures coverage matches specific cybersecurity challenges.
In regulatory compliance, AI automates checks and updates policies to meet evolving regulations, reducing manual tasks and ensuring accuracy. This proactive approach supports insurers in maintaining compliance and adapting swiftly to regulatory changes, fostering a culture of efficiency and adaptability.
Benefits of Generative AI in Insurance
In this section, we will delve into the advantages of harnessing generative AI in insurance, with a focus on enhanced risk assessment, streamlined claims processing, and personalized customer experiences.
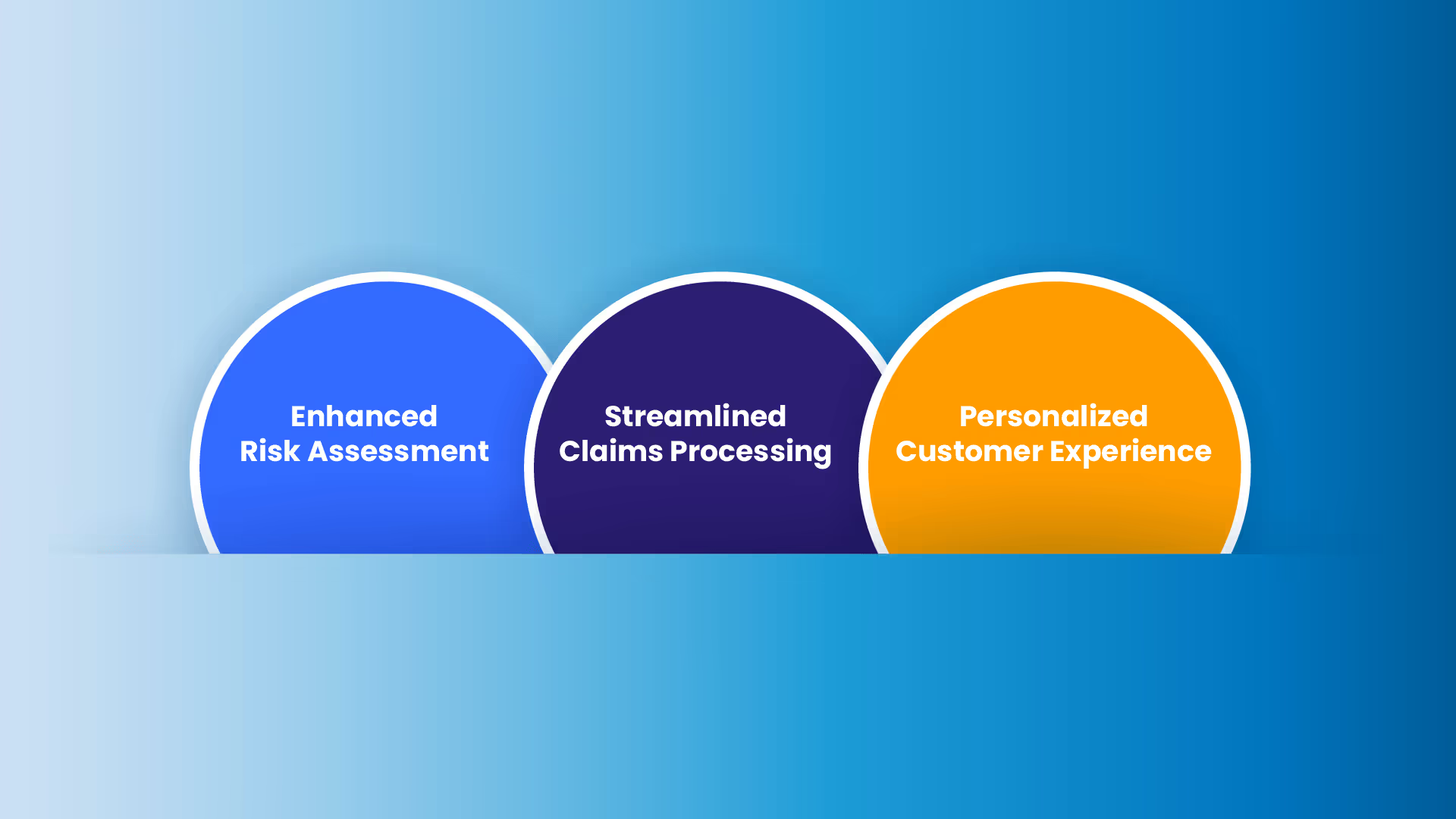
Enhanced Risk Assessment
Risk assessment lies at the heart of the insurance industry. Generative AI brings a new level of precision and sophistication to this critical task:
Granular Data Analysis: Generative AI has the capability to analyze vast and diverse datasets. By identifying hidden patterns and correlations within the data, it provides insurers with a deeper understanding of the risks associated with each policyholder.
Reduced Fraud
Fraudulent claims can be a significant cost for insurers. Generative AI can detect anomalies and unusual patterns in claim data, flagging potentially fraudulent activities. This proactive approach leads to substantial cost savings and maintains the integrity of the insurance pool.
Precise Pricing
Generative AI's ability to analyze multifaceted data sources enables insurers to determine policy prices with greater accuracy. This means that policyholders pay premiums that more closely align with their specific risk profiles, resulting in a win-win situation for both insurers and customers.
Consider an insurance company utilizing generative AI to analyze claims data. By identifying unusual patterns, such as a sudden increase in claims from a particular region, the AI system raises an alert. Investigating further, the insurer discovers a coordinated fraud scheme and takes immediate action, preventing substantial financial losses.
Additionally, generative AI can optimize pricing for auto insurance policies by analyzing telematics data, including driving behavior and vehicle conditions.
As a result, policyholders who drive safely and maintain their vehicles well enjoy lower premiums, while high-risk drivers pay rates commensurate with their risk level.
Streamlined Claims Processing
Claims processing is a critical aspect of insurance operations, and generative AI offers a powerful solution to expedite and enhance this process:
Efficiency and Speed
Generative AI-powered algorithms can process claims with remarkable efficiency, significantly reducing the time required for claim settlement. This not only enhances customer satisfaction but also frees up resources for insurers to allocate to more strategic tasks.
Error Reduction
Human errors in claim processing can lead to delays and disputes. Generative AI ensures consistency by consistently applying predefined criteria. This reduction in errors streamlines the entire claims process.
According to industry reports, insurance companies that have implemented AI-driven claims processing systems have achieved up to a 50% reduction in the time taken to settle claims. This streamlined process not only benefits policyholders by providing quicker payouts but also allows insurers to manage their operations more efficiently.
Personalized Customer Experience
Personalization is increasingly becoming a hallmark of excellent customer service, and the insurance industry is no exception:
Customer Retention
Tailoring policies and services to individual needs fosters stronger customer relationships. Policyholders who feel their insurance company understands and meets their specific requirements are more likely to remain loyal.
Cross-Selling and Upselling
Generative AI's insights into customer behavior and preferences empower insurers to identify opportunities for cross-selling additional coverage or upselling premium policies. This not only increases the average policy value but also ensures that customers receive the coverage they need.
Imagine a customer with a health insurance policy who is planning an international trip. Generative AI can analyze the customer's travel history, health data, and risk factors to customize an add-on policy that aligns perfectly with their unique requirements. This level of personalized service not only enhances customer satisfaction but also leads to increased policy sales and customer loyalty.
In the following sections, we will delve into practical implementation strategies for generative AI in these areas, providing actionable insights for insurance professionals eager to leverage this technology to its fullest potential.
Implementing Generative AI in Your Insurance Operations
In this section, we will guide insurance professionals through the process of implementing generative AI, including assessing your needs, choosing the right technology, and ensuring robust data management and privacy practices.
Assess Your Needs
Before diving headfirst into the world of generative AI, it's essential to conduct a thorough assessment of your organization's unique needs and pain points:
- Identify Operational Challenges: Start by identifying the specific areas within your insurance operations where AI could make a meaningful impact. This could be in underwriting, claims processing, customer service, or any other aspect.
- Set Clear Objectives: Define clear and measurable objectives for implementing generative AI. What do you aim to achieve? Whether it's reducing claims processing times, improving risk assessment accuracy, or enhancing customer experiences, having well-defined goals is essential.
- Assess Data Availability: Generative AI relies heavily on data. Evaluate the availability and quality of your data. Do you have access to the necessary data sources, or do you need to collect or enhance data?
- Budget Considerations: Determine your budget for AI implementation. This includes not only the cost of technology but also training, maintenance, and any necessary infrastructure upgrades.
Choosing the Right Technology
Selecting the right generative AI technology is a critical decision that can greatly impact the success of your implementation:
- Scalability: Consider the scalability of the technology. Will it be able to handle your growing data and processing needs as your organization expands?
- Integration: Ensure that the chosen technology can seamlessly integrate with your existing systems and workflows. Compatibility is key to a smooth implementation process.
- Accuracy and Performance: Evaluate the accuracy and performance of the AI technology. Look for real-world use cases and success stories to gauge its effectiveness.
- User-Friendliness: The technology should be user-friendly, enabling your team to work with it effectively. Intuitive interfaces and comprehensive training materials are essential.
- Support and Maintenance: Consider the level of support and maintenance required. Does the technology provider offer ongoing support, updates, and troubleshooting assistance?
If your organization lacks in-house AI expertise, it's highly advisable to seek consultation from AI experts or partner with AI solution providers. Experts can help you navigate the complexities of AI implementation, from selecting the right technology to fine-tuning algorithms and ensuring data security.
Data Management and Privacy
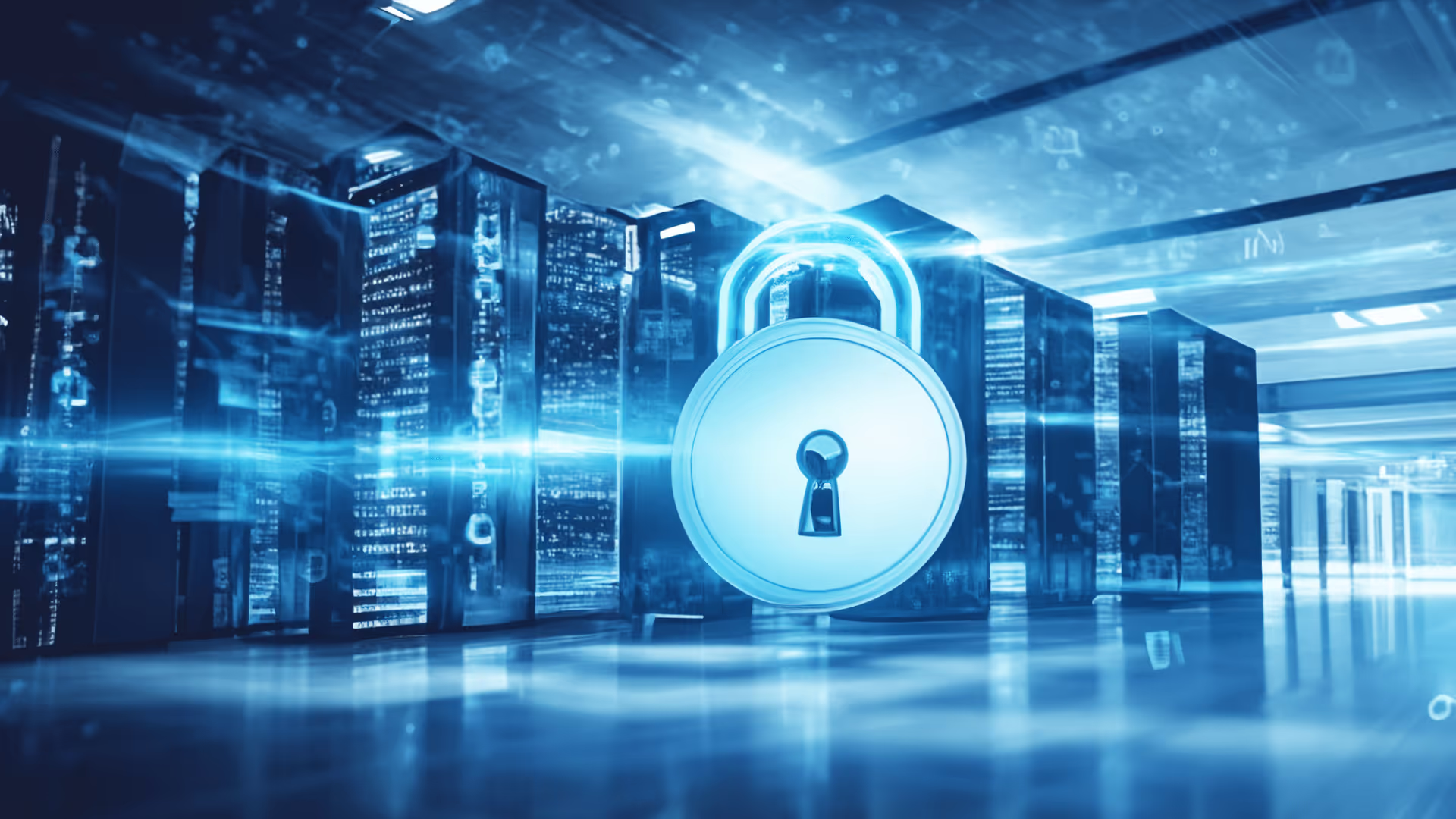
Data is the lifeblood of generative AI, making data security and compliance paramount:
- Data Encryption: Implement robust encryption protocols to protect sensitive customer data. Encryption ensures that even if data is breached, it remains unreadable and secure.
- Compliance: Familiarize yourself with data privacy regulations such as GDPR or HIPAA, depending on your location and the nature of your business. Ensure that your AI implementation is compliant with these regulations.
- Access Controls: Implement strict access controls to limit who can access and manipulate sensitive data. Ensure that only authorized personnel have access to AI systems and the data they utilize.
- Data Minimization: Collect only the data that is necessary for your AI applications. Avoid unnecessary data collection to minimize risk.
- Consent: Obtain clear and informed consent from customers regarding data usage. Communicate your data handling practices transparently.
- Regular Audits: Conduct regular data security audits to identify vulnerabilities and address them promptly.
- Employee Training: Train your employees in data security best practices, emphasizing the importance of protecting customer data.
By prioritizing data security and compliance and following responsible data handling practices, you can ensure that your generative AI implementation not only enhances your operations but also safeguards sensitive information.
Addressing Challenges in Implementing Generative AI for Insurance
The road to successful Generative Artificial Intelligence (AI) implementation in insurance may come with its fair share of challenges.
In this section, we'll explore common hurdles and provide strategies to overcome them, focusing on data quality and quantity challenges and the need for seamless integration with existing systems.
Data Quality and Quantity
High-quality data is the lifeblood of generative AI, but obtaining and maintaining it can be challenging:
- Data Availability: Insufficient data can hinder AI training and accuracy. In some cases, data may be scarce, particularly for emerging risks or niche markets.
- Data Quality: Data may be noisy, incomplete, or outdated, leading to inaccurate AI predictions. Inaccurate data can result in flawed risk assessments and pricing models.
- Data Augmentation: Augment your existing data with external sources, such as open data sets, to increase quantity and diversity. This can help improve the AI model's generalization.
- Data Cleansing: Implement data cleansing processes to identify and rectify errors, inconsistencies, and outliers. Regular data cleansing routines are essential to maintain data quality.
- Data Imputation: For missing data points, consider imputation techniques to estimate values based on existing data. However, use imputation cautiously, as it may introduce bias if not done correctly.
Integration with Existing Systems
Integrating generative AI with existing systems can pose compatibility and operational challenges:
- Legacy Systems: Legacy systems may lack the necessary interfaces to work seamlessly with AI solutions. This can lead to disjointed processes and inefficiencies.
- Operational Disruption: Poorly managed integration can disrupt day-to-day operations and hinder employee adoption of AI technologies.
- API-Driven Integration: Opt for API-driven integration approaches that allow AI systems to communicate with legacy systems. APIs enable data exchange and facilitate real-time decision-making.
- Gradual Implementation: Instead of a wholesale overhaul, consider a phased implementation strategy. Start with a specific use case or department, and gradually expand AI integration based on results and feedback.
- Employee Training: Invest in training programs for employees to familiarize them with AI tools and systems. Employee buy-in and proficiency are crucial for successful integration.
Successfully overcoming data quality and integration challenges is pivotal in realizing the full potential of generative AI in insurance. By addressing these obstacles strategically, you can ensure a smoother transition and maximize the benefits of AI implementation.
Measuring ROI and Continuous Improvement
The adoption of Generative Artificial Intelligence (AI) in insurance marks a significant investment, and ensuring a positive return on that investment is crucial.
This section will explore strategies for measuring ROI, including setting Key Performance Indicators (KPIs) and emphasizing the importance of continuous monitoring and optimization.
Setting KPIs
To gauge the success and impact of your generative AI initiatives, setting Key Performance Indicators (KPIs) is indispensable:
- Alignment with Objectives: KPIs align your AI efforts with your predefined objectives. Whether it's improving risk assessment accuracy, streamlining claims processing, or enhancing customer experiences, KPIs help measure progress toward these goals.
- Data-Driven Decision-Making: KPIs provide quantifiable metrics for data-driven decision-making. They allow you to assess the effectiveness of your AI systems objectively.
Relevant KPIs for insurance companies
- Claim Processing Time: Measure the time taken to process claims from submission to settlement. A decrease in this metric demonstrates improved efficiency.
- Accuracy of Risk Assessment: Track the accuracy of risk assessments made by AI systems compared to traditional methods. Higher accuracy indicates the value of AI in underwriting.
- Customer Satisfaction: Use customer surveys and feedback to measure satisfaction with AI-enhanced services. High satisfaction scores signify improved customer experiences.
- Fraud Detection Rate: Assess the percentage of fraudulent claims detected by AI systems. A higher rate indicates effective fraud prevention.
- Cost Savings: Calculate the cost savings achieved through AI implementation, including reduced administrative costs and fraud-related losses.
Regular Monitoring and Optimization
AI is not a set-it-and-forget-it solution. Continuous monitoring and optimization are essential for long-term success.
Here are a few steps to ensure regular monitoring and optimization:
- Ongoing Evaluation: Regularly evaluate the performance of your AI models against established KPIs. Identify areas where improvements are needed.
- Fine-Tuning: Based on performance data, fine-tune AI models to enhance accuracy and efficiency. Adjust algorithms, data sources, and parameters as necessary.
- Feedback Loop: Create a feedback loop that incorporates insights from employees and end-users. Their feedback can provide valuable information for optimization.
Periodic evaluations and adjustments.
- Quarterly Reviews: Conduct quarterly reviews of AI performance and KPIs. Use these reviews to identify trends and make informed decisions about adjustments.
- Adaptive Strategies: Be prepared to adapt your AI strategies based on changing business needs, emerging risks, and evolving technology.
Conclusion
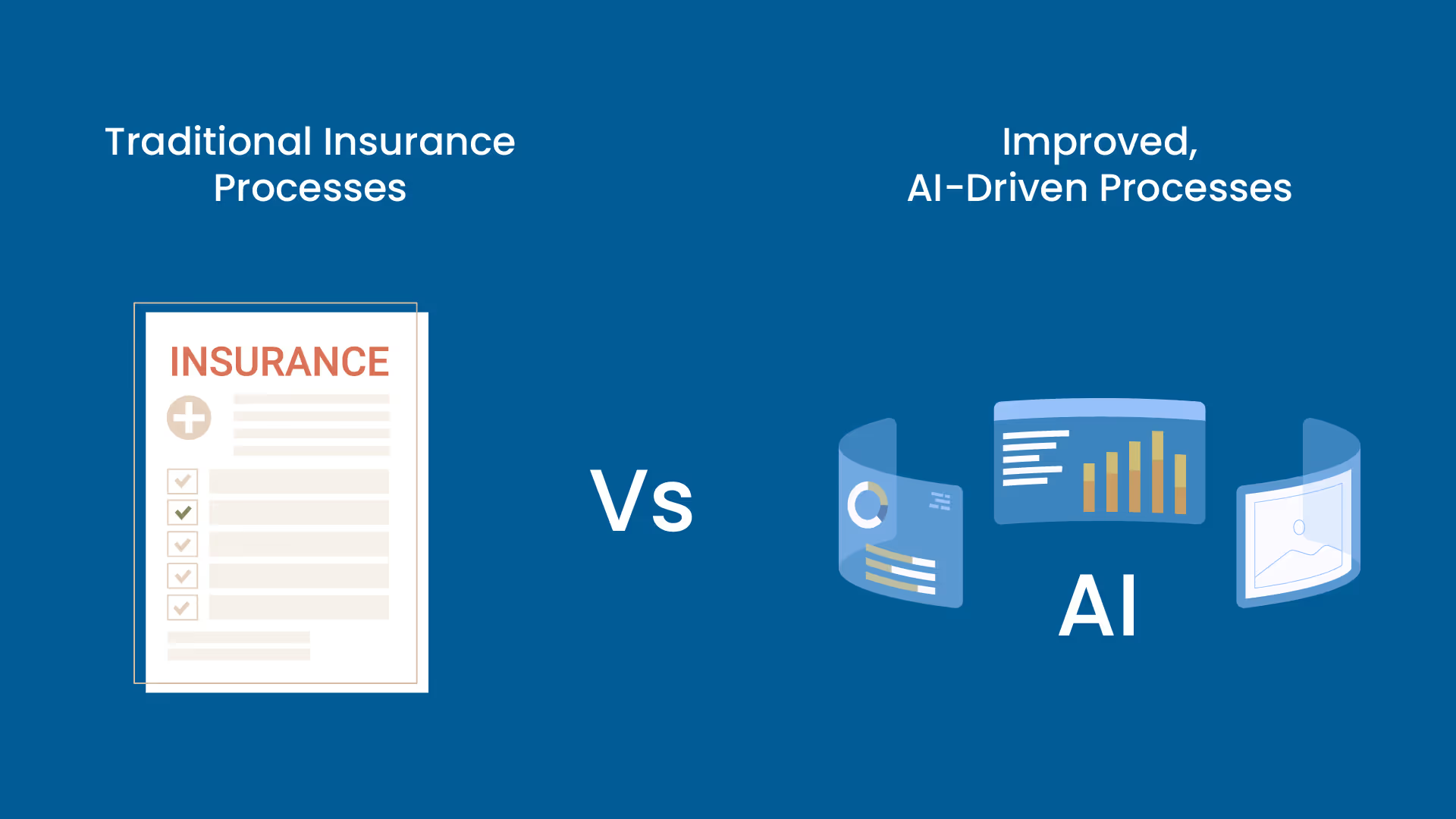
In conclusion, generative AI holds immense potential to revolutionize the insurance industry. From enhanced risk assessment to streamlined claims processing and personalized customer experiences, the benefits are substantial.
However, successful implementation requires careful planning, addressing data quality challenges, and seamless integration with existing systems.
Setting clear KPIs is essential to measuring the impact of generative AI on your insurance operations.
Regular monitoring and optimization ensure that AI systems continue to deliver value and adapt to changing circumstances.
We encourage insurance professionals to embrace generative AI as a competitive edge in an increasingly dynamic and data-driven industry.
By leveraging AI's capabilities and continually refining your strategies, you can stay ahead of the curve and provide superior services to your policyholders.
Transform your insurance business with Ideas2IT's Generative AI. Enhance efficiency, accuracy, and customer-centricity. Learn how our AI solutions can give you a competitive edge in the industry.