Generative Artificial Intelligence (AI) has evolved from its theoretical roots to become a formidable force, profoundly reshaping the healthcare landscape. The application of Generative AI in healthcare is more than just a futuristic concept; it's a tangible reality backed by compelling data.
According to market analysis firm, Grand View Research's Healthcare AI Market Size, Share & Trends Analysis Report 2021, the global healthcare AI market has already reached a value of $6.6 billion, with a projected annual growth rate of 41% between 2022 and 2028.
This meteoric rise is indicative of the transformative potential that Generative AI holds for the industry.
Harnessing the capabilities of Generative AI, we are now entering an era marked by remarkable advancements in healthcare. These advancements are poised to drive precision medicine, streamline operational efficiency (scheduling), and elevate the standard of patient-centric care to unprecedented levels.
With that being said, let us explore the possibilities in Healthcare with Generative AI being incorporated into it.
The Practical Applications of Generative AI in Healthcare

Generative AI, a subset of artificial intelligence, empowers machines to create and generate data that closely resembles human-generated content. In the realm of healthcare, this technology is poised to make a profound impact, and the data supports its potential.
Generative AI has already demonstrated its prowess in various healthcare domains.
For instance, in medical imaging, Generative Adversarial Networks (GANs) have shown impressive results. A study published in the journal 'Nature Medicine' reported that GANs improved diagnostic accuracy in breast cancer mammography by over 5.7%.
This data underscores how Generative AI is enhancing the precision of medical imaging, potentially saving lives through early and more accurate diagnoses.
Moreover, the pharmaceutical industry is also experiencing a transformation driven by Generative AI. The industry is expected to save over $70 Billion annually by optimizing drug discovery processes with the help of Generative AI. This not only improves efficiency but also accelerates the development of life-saving drugs.
For patient data analysis, Generative AI is helping healthcare providers by processing vast datasets and assisting in identifying disease trends, predicting patient outcomes, and personalizing patient treatment plans.

1. Medical Image Generation and Enhancement
Generative AI, leveraging techniques such as Generative Adversarial Networks (GANs) and Variational Autoencoders (VAEs), has emerged as a transformative tool in the realm of medical imaging. One of its notable applications is the generation of high-resolution medical images, including MRI and CT scans.
This capability is invaluable, as acquiring such high-quality images traditionally involves costly and sometimes invasive procedures, making generative AI a cost-effective and patient-friendly alternative.
By utilizing generative AI for MRI and CT scan synthesis, medical professionals can access synthetic images that closely mimic real scans. These synthetic images serve various purposes, from educational and research needs to training machine learning models. This diminishes the reliance on extensive, often scarce, and ethically challenging data collection, potentially accelerating advancements in healthcare.
Moreover, generative AI's proficiency extends to the enhancement of existing medical images. In instances where acquired images suffer from inherent issues like noise, motion artifacts, or low resolution, generative models come to the rescue.
They can be trained to remove noise and artifacts, a process particularly crucial for accurately interpreting medical conditions. In noisy environments like emergency rooms, or when patients exhibit involuntary motion during scans, these models ensure clearer and more reliable images.
Furthermore, the application of super-resolution techniques is paramount in the medical field. Generative AI can upscale low-resolution medical images, enhancing them to higher resolutions while retaining critical anatomical details.
This technology proves indispensable in scenarios where older imaging equipment or limited resources necessitate image improvement. It enables healthcare providers to make more informed decisions regarding patient care.
The impact of generative AI on healthcare goes beyond image enhancement; it significantly contributes to diagnosis and treatment planning. High-quality, noise-free images facilitate precise diagnosis by medical professionals. They can more accurately identify and evaluate abnormalities, tumors, lesions, or subtle changes in patient scans.
Consequently, generative AI plays a pivotal role in minimizing diagnostic errors and improving patient outcomes.
In the realm of treatment planning, generative AI-driven image improvement is equally beneficial. Surgeons, oncologists, and other specialists rely on clear and detailed medical images to strategize and execute interventions effectively. By providing the necessary image quality, generative AI becomes an indispensable tool in optimizing medical procedures.
Additionally, synthetic medical images generated by AI find utility in medical education and training. These images enable medical students and professionals to hone their diagnostic skills, offering a safe and ethical alternative to real patient data for practice and refinement.
2. Natural Language Processing (NLP) for Electronic Health Records (EHR)
NLP (Natural Language Processing) models driven by generative AI have emerged as powerful tools in the healthcare sector, particularly in managing electronic health records (EHRs). These models excel at deciphering unstructured EHR data, which often includes free-text clinical notes, physician narratives, and other textual information. By doing so, they enable healthcare providers to unlock a wealth of valuable information. This transformative capability has several profound implications for the healthcare industry.
First and foremost, NLP-powered generative AI facilitates quick and efficient access to patient data. EHRs typically contain vast amounts of information, and manually sifting through them can be time-consuming and error-prone. NLP models can swiftly extract relevant data, such as patient histories, treatment plans, and medication records, enabling healthcare professionals to make more informed decisions promptly. This not only enhances patient care but also contributes to overall operational efficiency within healthcare institutions.
Furthermore, the integration of NLP into EHR systems streamlines administrative tasks. These models can assist in automating processes like patient documentation, note-taking, and data entry, allowing healthcare providers to allocate more time to direct patient care. By reducing the administrative burden, NLP helps improve the productivity and job satisfaction of healthcare professionals.
Another significant benefit is the enhanced accuracy of medical coding and billing. Accurate coding is crucial for proper reimbursement and compliance with healthcare regulations. NLP models can assist in extracting and categorizing clinical information, ensuring that the appropriate billing codes are assigned. This reduces billing errors, minimizes revenue loss, and helps healthcare facilities maintain financial stability.
3. Drug Discovery and Molecular Modeling
Foremost, generative AI is revolutionizing the pace of drug discovery. In the traditional drug development pipeline, the identification and optimization of drug candidates involve laborious experimental testing and screening, which can span many years.
However, generative AI can rapidly generate and evaluate an expansive array of molecular structures in a virtual environment, compressing the timeline for identifying promising compounds. This acceleration is especially pivotal in addressing urgent medical needs, such as emerging health crises, where swift solutions are imperative.
Beyond expediting drug discovery, generative AI contributes to substantial cost savings. Pharmaceutical research is inherently resource-intensive, demanding extensive laboratory work and vast datasets.
By automating key aspects of the drug development process, generative AI reduces the need for resource-intensive experiments and accelerates decision-making. Consequently, it significantly lowers research costs, making drug development more economically viable for pharmaceutical companies and research institutions.
One of the most promising facets of generative AI is its capacity to foster innovation in drug design.
By analyzing diverse datasets encompassing chemical information and biological data, these models can suggest novel drug candidates with unique properties and mechanisms of action. This groundbreaking approach opens up new possibilities for addressing previously deemed intractable diseases. It offers hope for rare or orphan diseases, which often face limited research attention, by facilitating the discovery of novel therapeutic avenues.
4. Personalized Treatment Plans and Predictive Analytics
Generative AI represents a groundbreaking frontier in healthcare by leveraging a patient's comprehensive health profile, including medical history, genetic data, and real-time health metrics, to craft highly personalized treatment plans.
This transformative capability empowers healthcare providers to offer individualized care that factors in a patient's unique genetic makeup, past medical experiences, and current health status.
By mining this wealth of information, generative AI can recommend treatments tailored to the patient's specific needs, optimizing efficacy and minimizing potential side effects. This personalization not only enhances patient outcomes but also improves the overall efficiency of healthcare delivery.
Moreover, generative AI extends its impact by predicting disease progression and identifying individuals at elevated risk.
By analyzing historical health data and genetic predispositions, these models can forecast how a disease may evolve, providing valuable insights for early intervention and treatment adjustment.
Identifying high-risk patients allows healthcare systems to proactively allocate resources and interventions, ultimately leading to more effective management of chronic conditions, reduced healthcare costs, and, most importantly, improved patient well-being.
In essence, generative AI's ability to combine personalized treatment planning with predictive analytics is revolutionizing healthcare by ushering in an era of proactive and patient-centered medicine.
5. Medical Chatbots and Virtual Assistants
Virtual healthcare assistants powered by generative AI can engage with patients through natural language conversations. They can provide information, schedule appointments, offer medication reminders, and even triage patients by assessing their symptoms, enhancing patient engagement and accessibility to healthcare services.
6. Healthcare Resource Optimization
Generative AI aids healthcare by facilitating resource optimization by predicting patient admission rates, staff scheduling, and inventory management, leading to more efficient and cost-effective operations.
7. Drug Interaction Prediction:
Generative AI models can analyze large datasets of drug interactions to predict potential drug-drug interactions and adverse reactions. This information helps healthcare providers make informed decisions when prescribing multiple medications to a patient.
8. Genomic Data Analysis:
Generative AI can assist in analyzing vast amounts of genomic data, identifying genetic markers associated with specific diseases, and aiding in personalized medicine approaches. This has implications for cancer treatment, genetic counseling, and rare disease diagnosis.
Medi Tutor
"Medi Tutor" consolidates research reports, papers, medical news, and hospital records, offering an intuitive app with chat and voice interfaces.
It generates synthetic scenarios and study materials, tailored to physicians, nurses, technicians, and other healthcare personnel, to enhance their skills and knowledge.
The benefits of "Medi Tutor" are profound, including easier continuous education, familiarity with best practices, enhanced competency, and improved patient care.
In the dynamic world of healthcare, "Medi Tutor" ensures that healthcare professionals remain well-informed, confident, and ready to deliver high-quality care, ultimately leading to improved patient outcomes.
Virtual Wellness Coach
In the journey towards better health and well-being, a Virtual Wellness Coach serves as an invaluable companion.
This digital wellness partner offers personalized guidance on nutrition, exercise, stress management, and overall health improvement. It stands as a motivating force, tailored to individuals' specific needs and goals.
The implementation of a Virtual Wellness Coach comes with a multitude of benefits. Individuals find themselves empowered to make positive lifestyle changes, creating healthier habits and ultimately leading to improved well-being.
Beyond personal growth, this innovation also translates to reduced healthcare costs and contributes to the overall health and vitality of the population.
Appointment Scheduler
In the healthcare landscape, traditional appointment scheduling often poses challenges for both patients and providers.
Generative AI introduces an innovative solution through a conversational interface that streamlines the appointment booking process.
This technology not only lightens the workload for front desk personnel but also ensures that patients can access the scheduling system around the clock, eliminating the need for extended hold times.
Patients receive valuable suggestions and reminders, leading to improved preparedness for their appointments. Furthermore, providers can efficiently manage appointments, resulting in increased patient satisfaction and a more streamlined healthcare experience.
Utilization of Generative AI by Private Payers
- Healthcare management
- Member services
- Provider relationship management
- Corporate functions
- Claims management
- Marketing and sales
- Fraud Detection
Addressing Healthcare Challenges with Generative AI
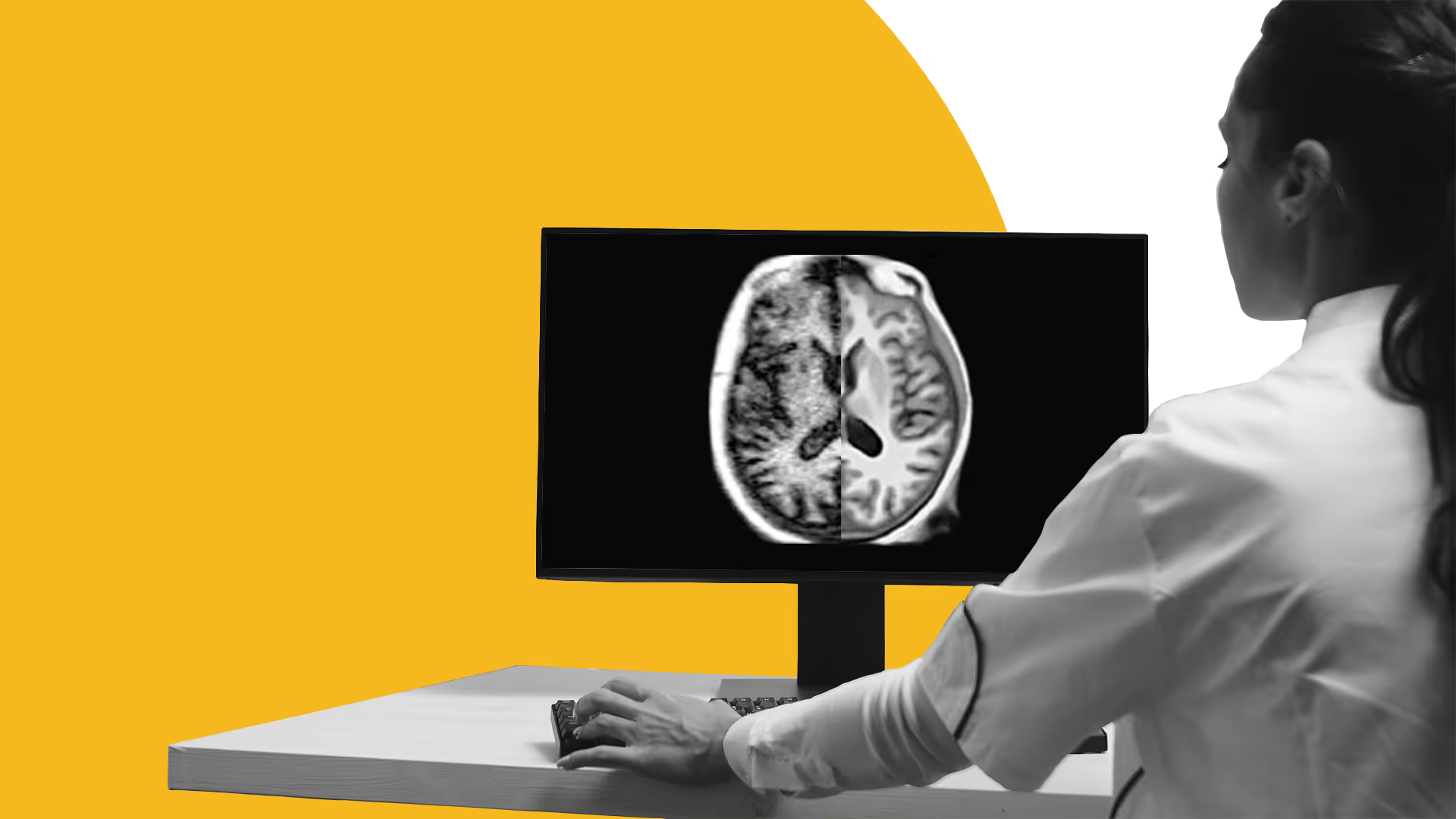
Consider the last time you encountered a challenge within the healthcare system – perhaps it was the time-consuming process of diagnosing complex medical conditions, the painstaking search for effective drug candidates, or even the struggle to provide personalized treatment recommendations for each patient.
These are the persistent pain points that healthcare professionals and organizations wrestle with daily, and Generative AI is emerging as a compelling solution.
For instance, in medical imaging, Generative Adversarial Networks (GANs) have demonstrated their ability to improve the quality of magnetic resonance images (MRIs) and computed tomography (CT) scans, leading to more accurate diagnoses.
Furthermore, in the realm of patient data analysis, the use of Generative AI has allowed specialists to achieve AI-driven analysis of electronic health records, leading to more personalized treatment recommendations.
This personalized approach addresses the challenges associated with deriving insights from the vast and complex data generated within healthcare systems.
Generative AI is not merely a temporary fix but a transformative solution poised to reshape the entire healthcare landscape. By harnessing the power of AI to generate data, images, and insights, Generative AI presents a unique opportunity to address the long-standing limitations of the healthcare industry.
Imagine medical images that are not only clearer but also enhanced, aiding healthcare professionals in making swift and accurate diagnoses. Visualize patient data analysis powered by AI, leading to personalized treatment plans that consider individual characteristics and needs.
Challenges and Ethical Considerations

Navigating the landscape of Generative AI in healthcare involves addressing crucial challenges and ethical considerations.
As this technology evolves, it's essential to ensure data security, mitigate biases, and maintain transparency for responsible and impactful healthcare innovation.
1. Data Privacy and Security
The importance of data privacy and security was underscored by data breaches and cyber threats in the healthcare sector.
According to a report by Protenus, healthcare data breaches affected over 9.3 million patient records in 2020, highlighting the vulnerability of patient information.
Patient information is exceptionally sensitive, encompassing details that must be strictly protected. The incorporation of AI introduces complexities surrounding unauthorized access to this information.
As Generative AI delves into analyzing and generating insights from these datasets, there's a critical need to establish robust data security measures. This involves implementing strong encryption protocols, access controls, and stringent authorization mechanisms.
2. Bias in AI Algorithms
While the capabilities of AI are transformative, they also come with the challenge of potential bias ingrained within algorithms. Biases present in historical data can inadvertently influence AI-generated solutions, resulting in inaccuracies and unfair outcomes.
In healthcare, this bias can lead to incorrect diagnoses or treatment recommendations. It's essential to address these biases proactively, beginning with an extensive evaluation of training data sources to identify and rectify any existing biases.
Implementing fairness-aware algorithms and ongoing monitoring can help mitigate the impact of bias and enhance the accuracy and equity of AI-generated insights.
3. Transparency and Accountability
Transparency is a cornerstone of ethical AI application in healthcare decision-making. As AI becomes increasingly intertwined with healthcare practices, healthcare professionals must comprehend how AI reaches its conclusions.
Generative AI service providers need to embrace transparency initiatives, ensuring that the decision-making process of AI models is understandable and traceable.
This involves explaining the factors that contribute to AI-generated outputs, making it possible for healthcare experts to trust and validate these insights.
By prioritizing transparency, the healthcare industry can foster accountability and ensure that AI complements human expertise rather than replacing it.
Future Implications and Possibilities
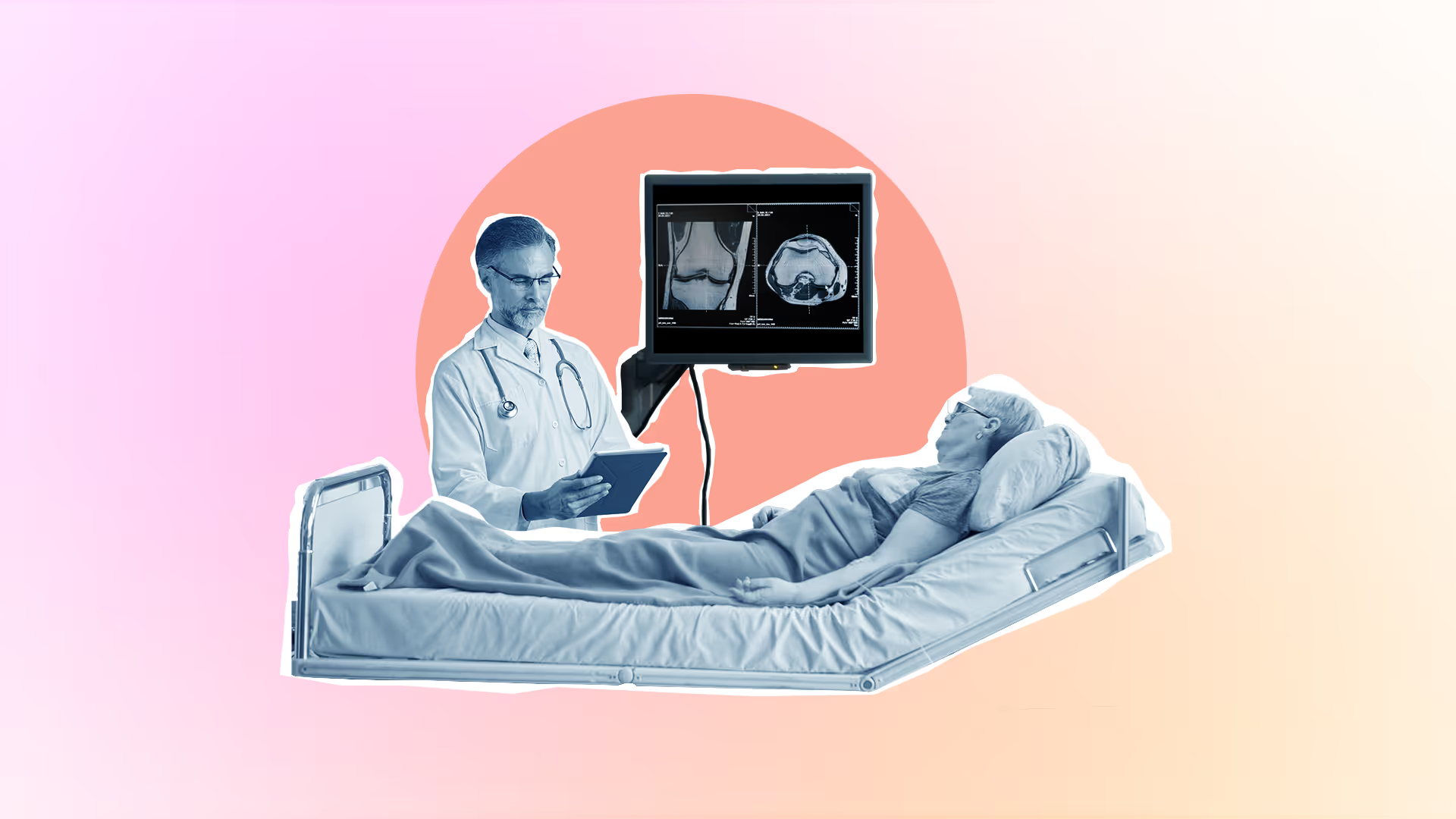
The integration of Generative AI into healthcare is poised to unlock a realm of transformative possibilities, revolutionizing how we approach diagnostics, treatment planning, and the collaboration between AI and medical professionals.
1. Enhanced Diagnostics and Treatment Planning
Imagine a future where medical diagnoses are not just accurate but also rapid. Generative AI has the potential to revolutionize diagnostics by quickly analyzing medical data, images, and symptoms to provide precise insights. With AI-assisted diagnostics, healthcare professionals can make informed decisions faster, leading to timely treatments and improved patient outcomes.
2. AI-Augmented Medical Professionals
The partnership between AI and medical professionals is set to redefine healthcare practices. AI can assist medical practitioners by processing vast amounts of data, recognizing patterns, and suggesting potential diagnoses or treatment plans. This augmentation allows healthcare professionals to focus on patient care, while AI handles the data-intensive aspects, resulting in more efficient and comprehensive healthcare delivery.
3. Collaborative AI-Human Healthcare Ecosystem
The future of healthcare is collaborative – a harmonious synergy between AI and human expertise. Imagine a scenario where AI-driven insights complement the knowledge and experience of medical professionals. This collaborative ecosystem ensures that patients receive the best of both worlds: the precision of AI and the empathy and judgment of healthcare providers.
Enhance your healthcare practice with Generative AI
Generative AI algorithms are advancing at a rapid pace. While AI holds tremendous potential, its misuse can have significant consequences, especially in healthcare. Organizations must approach AI deployment cautiously.
Here are three essential tips for healthcare organizations considering AI-based solutions:
- Facilitate employee adoption: Human oversight remains essential, especially in healthcare's regulated environment. Implement a human-in-the-loop approach where staff supervise AI models. Provide comprehensive training to medical and administrative personnel on AI integration. Encourage employees to adapt their workflows to incorporate AI efficiently, enabling them to focus on higher-value tasks with the time saved.
- Take control of AI models: Embrace responsible AI practices. Clarify when and how to use AI tools, and designate accountability for outcomes. Begin with pilot projects of low-impact use cases to test generative AI models before scaling to more critical applications. Understand that while generative AI can be highly accurate, errors can occur. Define acceptable error rates based on application sensitivity—higher accuracy standards are crucial for diagnostic and patient-facing roles than administrative tasks.
- Prepare your data: Data preparation is critical. Even when using pre-trained AI models, retraining them on your specific dataset—ensuring it's high-quality and representative of your patient population—is advisable. Secure medical data rigorously to protect patient privacy. Transparency about the training dataset helps understand AI performance strengths and limitations.
By following these guidelines, healthcare organizations can harness the transformative potential of generative AI responsibly. This approach ensures improved operational efficiency, enhanced patient care, and compliance with regulatory standards, paving the way for successful AI integration in healthcare settings.
As we conclude this journey into the realm of Generative AI in healthcare, one thing becomes abundantly clear: the future of healthcare is here, and it's brimming with promises.
The integration of Generative AI has the power to reshape diagnostics, treatment planning, and the very nature of healthcare collaboration.
What was once considered science fiction is now a tangible reality, ready to transform patient care as we know it. From the speed and efficiency of analysis to the promise of AI-augmented medical professionals, we're standing on the cusp of a new era.
Challenges like data privacy, bias, and transparency are not insurmountable obstacles but rather stepping stones toward a more ethical and responsible application of AI in healthcare.
In the convergence of Generative AI and healthcare, a new era of innovation and patient-centricity dawns – a future where possibilities are limitless, and healthcare excellence is redefined.