In today's landscape of banking and finance, Generative Artificial Intelligence (Gen AI) has emerged as a game-changing catalyst for transformation. Far beyond traditional data processing, Generative AI possesses the remarkable ability to generate insights, solutions, and opportunities that are redefining the financial sector.
In this insightful blog, we will explore seven compelling use cases that vividly demonstrate how Generative AI is beneficial to the banking industry.
From revolutionizing credit risk assessments to deploying intelligent chatbots for unparalleled customer service and bolstering security with real-time fraud detection, Generative AI is actively redefining the operational paradigms of banks.
We'll also dive into the intricate ways Gen AI optimizes trading strategies, personalizes marketing efforts, and fortifies Anti-Money Laundering (AML) practices, providing a comprehensive overview of its multifaceted impact.
Additionally, we’ll also explore the pivotal challenges and ethical considerations that come hand-in-hand with this AI-driven transformation.
With that said, let’s get right in.
Use Case 1: Credit Risk Assessment
In banking, assessing credit risk is a fundamental process that determines the lending decisions institutions make.
Traditionally, credit risk assessment relied on historical data and statistical models. However, generative AI brings a new level of precision and predictive power to this process. By analyzing vast datasets and generating sophisticated credit scoring models, it can evaluate an applicant's creditworthiness more accurately than ever before.
Gen AI takes into account a wide range of factors, including transaction history, social data, and economic indicators. It can identify subtle patterns and correlations that human analysts might miss, ultimately reducing default risks and improving loan approval rates.
The impact is significant. Banks can make lending decisions faster and with greater confidence. It enables them to offer loans to a broader spectrum of customers, including those who may have been previously overlooked or considered too risky.
Use Case 2: Chatbots for Customer Service
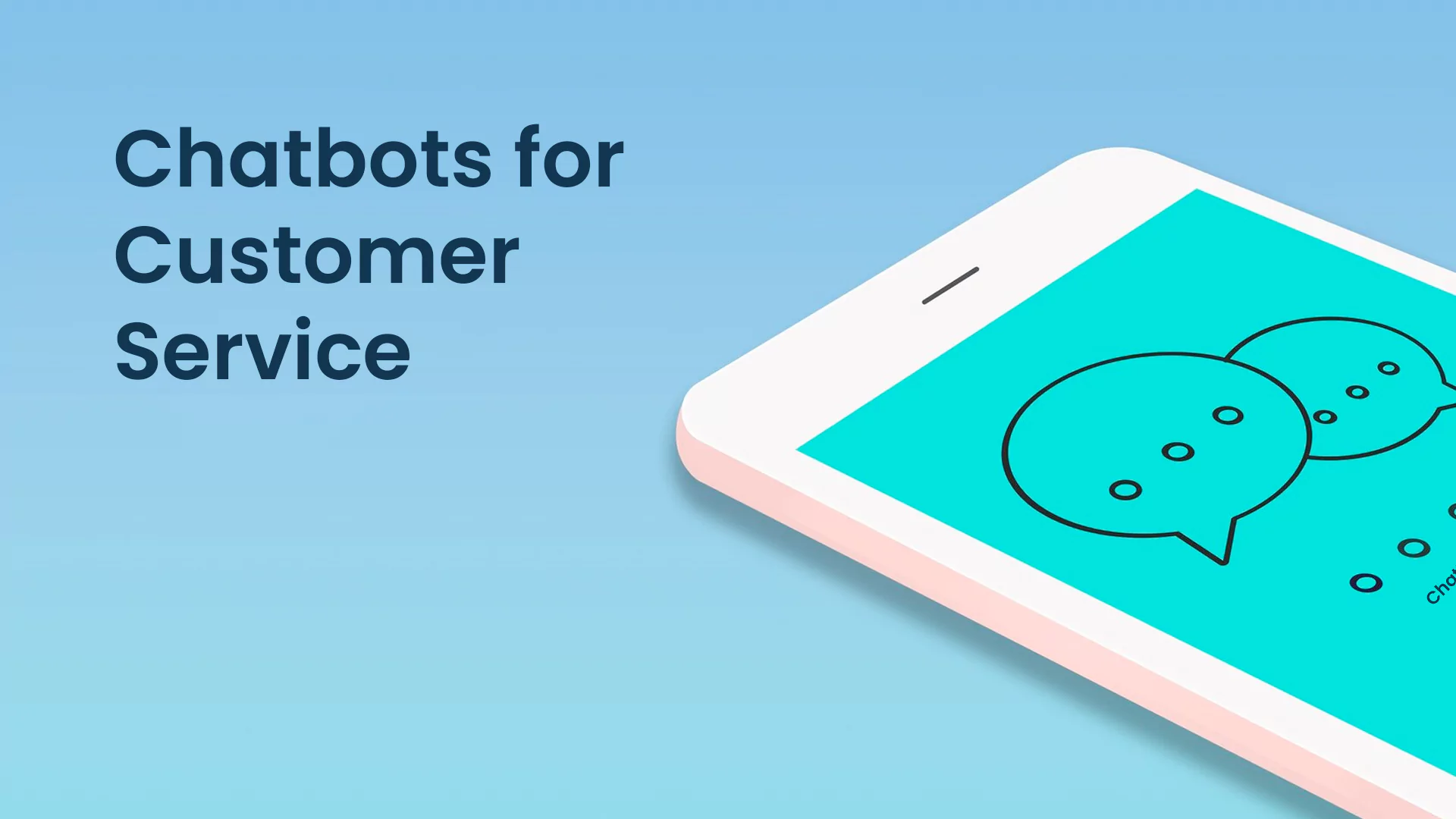
Customer service has always been a cornerstone of success. However, serving the diverse needs of customers efficiently and effectively can be a challenge. This is where Generative AI-powered chatbots step in.
Generative AI-driven chatbots can engage customers in natural, human-like conversations, providing instant assistance 24/7. These bots are not just rule-based; they understand context, sentiment, and nuances in language, making interactions seamless and personalized.
When a customer has a query or needs assistance, the chatbot uses generative AI to analyze the inquiry and provide relevant responses or solutions. Whether it's checking account balances, explaining transaction details, or helping with account setup, these chatbots can handle a wide range of tasks, freeing up human agents to focus on more complex issues.
Using this, banks can enhance customer satisfaction by offering round-the-clock support, reducing operational costs, and improving response times. Furthermore, chatbots can collect valuable customer data, enabling banks to better understand their clientele and tailor services accordingly.
Generative AI-driven chatbots are becoming the new face of customer service in banking, enhancing the overall experience for customers while boosting operational efficiency.
Use Case 3: Fraud Detection
The battle against financial fraud has taken on new dimensions with the integration of Generative AI in the banking sector. Detecting and preventing fraudulent activities in real-time is crucial to maintaining trust and security within the financial ecosystem.
Generative AI-driven fraud detection systems are designed to constantly monitor transactions and identify irregularities. These systems employ machine learning models that not only analyze historical transaction data but also generate predictive models to detect fraudulent patterns as they evolve.
What sets Generative AI apart in this use case is its adaptability. It learns from new data and adjusts its fraud detection algorithms accordingly, making it highly effective against both known and emerging threats. Moreover, it reduces false positives, ensuring that legitimate transactions are not mistakenly flagged as fraudulent.
Banks can thus benefit significantly from Generative AI-powered fraud detection. It helps prevent financial losses, protects customers from unauthorized transactions, and maintains the institution's reputation.
Use Case 4: Algorithmic Trading
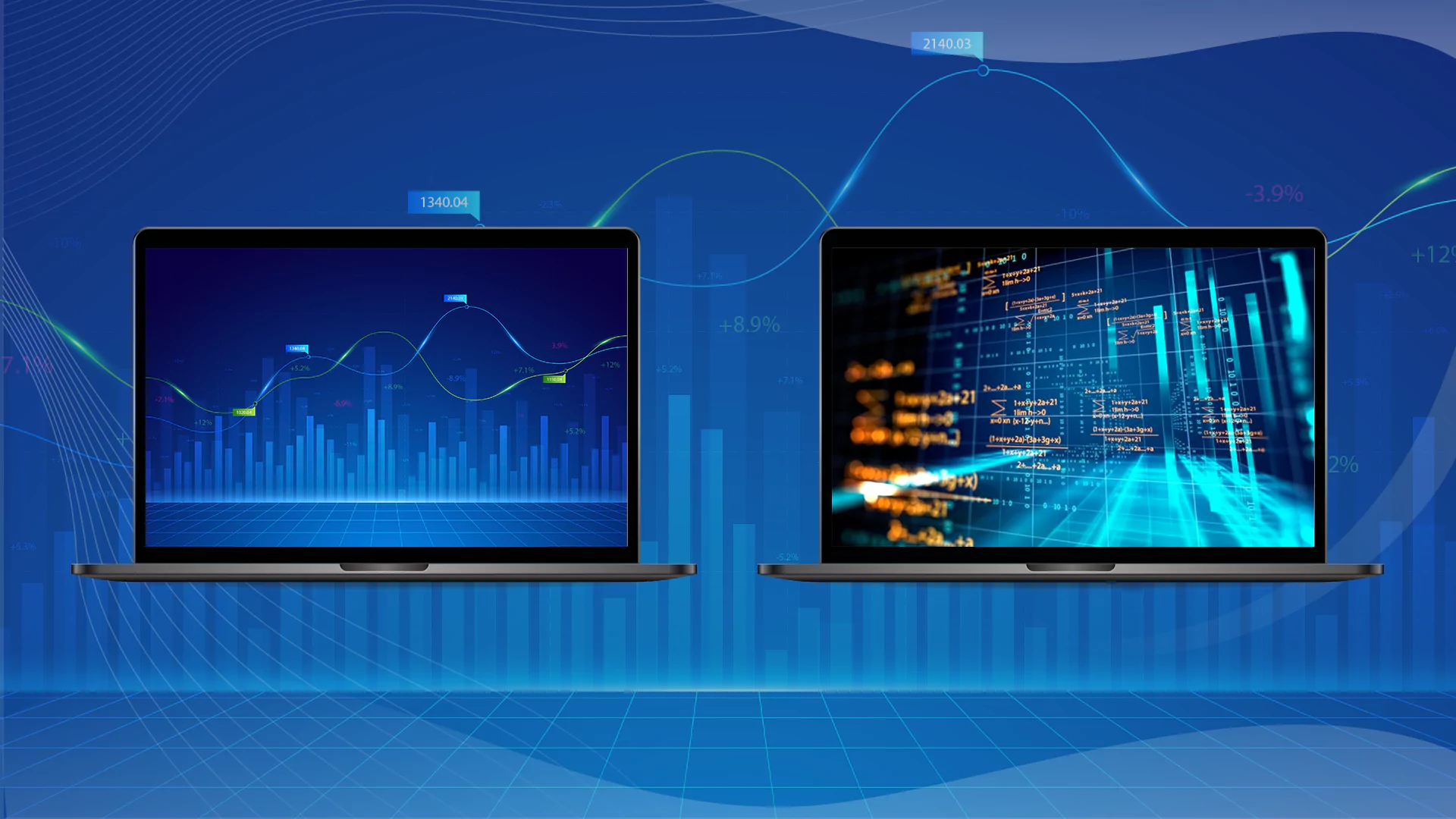
Algorithmic trading has become a cornerstone of modern finance, and Generative AI is at the heart of its evolution. Banks and financial institutions rely on AI-driven trading strategies to optimize their investments and stay competitive in the fast-paced world of financial markets.
Generative AI models analyze vast amounts of market data, historical trading patterns, news sentiment, and even social media trends. These models then generate sophisticated algorithms that can make split-second trading decisions based on the insights derived from this data.
Generative AI shines in algorithmic trading thanks to its adaptability and ability to learn. These models continuously update themselves, allowing them to react to changing market conditions and emerging trends with precision. This results in more efficient trading strategies that can maximize returns and minimize risks.
The impact on banks is substantial. They can execute trades with unparalleled speed and accuracy, improving their market position and profitability. Algorithmic trading powered by Generative AI also allows for the exploration of new trading strategies that were previously unimaginable.
In a world where milliseconds can make a difference, Generative AI has become a crucial tool for financial institutions seeking to gain an edge in the highly competitive landscape of algorithmic trading.
Use Case 5: Gen AI Chatbots for Personalized Marketing in Banking
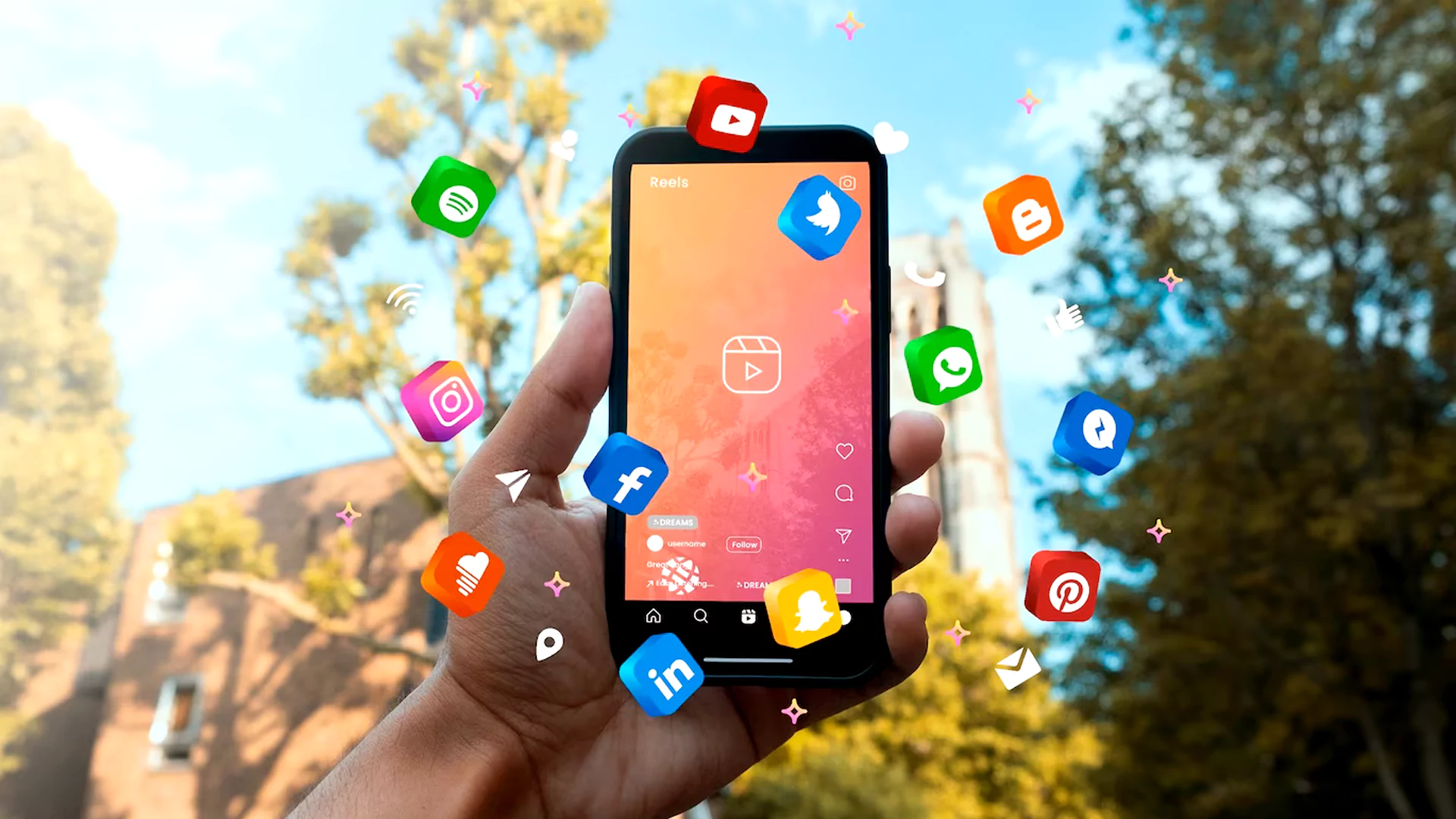
Generative AI models can analyze vast amounts of customer data, including transaction history, browsing behavior, and demographic information. Using this data, AI can generate highly personalized marketing campaigns and product recommendations tailored to individual customers.
This personalization extends beyond generic email offers. Gen AI can craft targeted messages, content, and even product offerings that resonate with each customer's preferences and needs. This level of customization not only enhances customer engagement but also drives conversion rates and customer loyalty.
For banks, the benefits are clear. Personalized marketing powered by Generative AI can lead to higher customer satisfaction, increased cross-selling opportunities, and a more significant return on marketing investments. Banks can deliver the right product or service to the right customer at the right time.
Use Case 6: Wealth Management and Portfolio Optimization
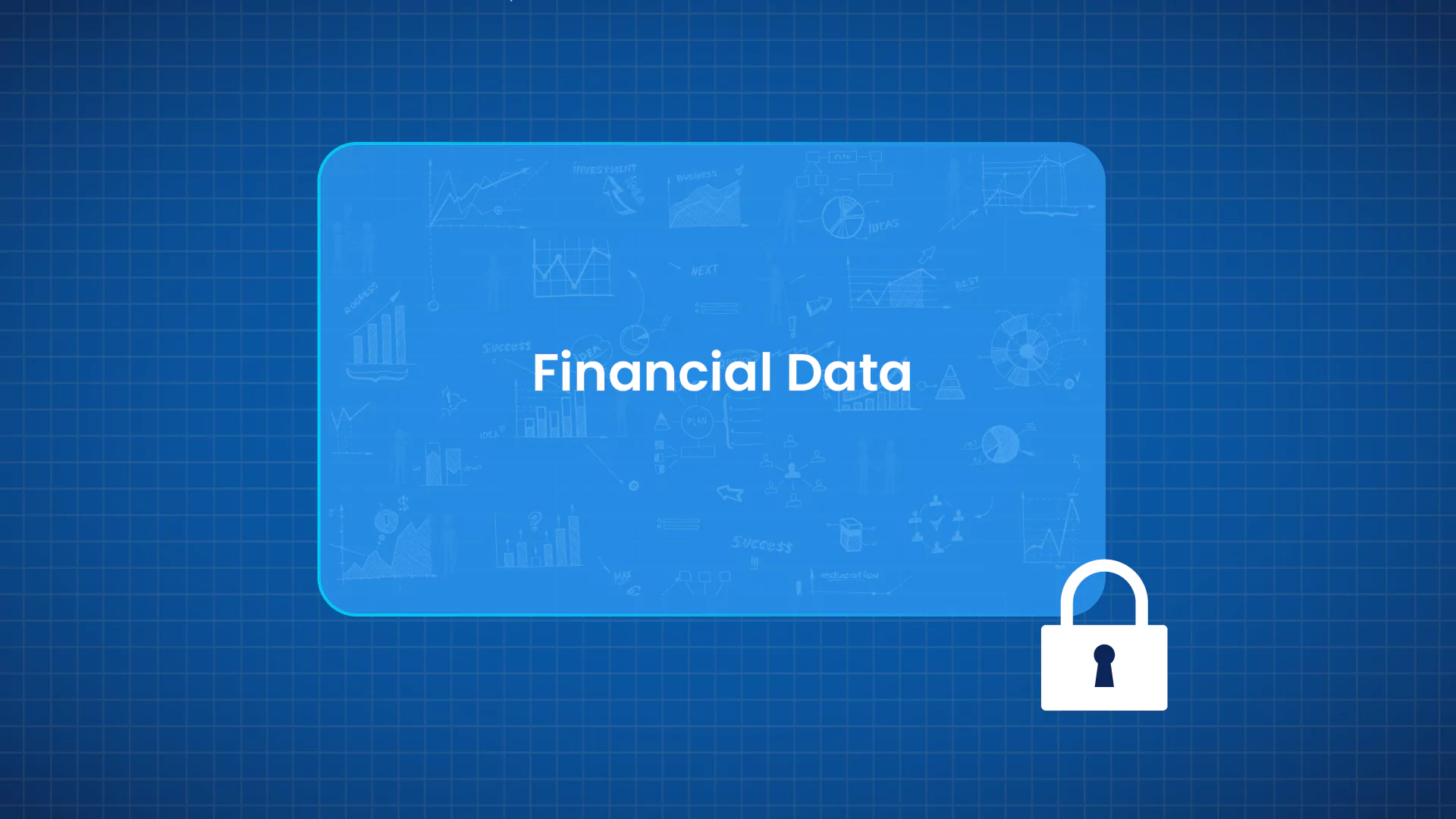
Wealth management is a critical area in banking, where clients entrust financial institutions to grow and safeguard their assets. Generative AI is playing a pivotal role in enhancing wealth management and portfolio optimization processes.
Generative AI models can analyze a vast array of financial data, economic indicators, market trends, and individual client profiles. Using this data, AI can generate predictive models that recommend optimal asset allocations and investment strategies.
These models can adjust portfolios in real-time based on changing market conditions and emerging opportunities. This dynamic approach to wealth management allows banks to maximize returns while managing risk effectively.
For banks, the advantages are substantial. Wealth managers can provide clients with more personalized investment strategies and asset allocations, leading to improved client satisfaction and loyalty. Additionally, AI-driven wealth management can reduce operational costs and increase the scalability of services.
Use Case 7: Anti-Money Laundering (AML)
Preventing money laundering and complying with regulatory requirements is a paramount concern for banks. Generative AI is proving to be a formidable ally in enhancing Anti-Money Laundering (AML) practices.
Generative AI models can analyze massive volumes of transaction data, customer profiles, and historical patterns to identify suspicious activities. These models not only detect known money laundering techniques but also adapt to evolving schemes, ensuring banks stay ahead of criminal tactics.
What makes Generative AI particularly effective in AML is its ability to generate predictive models that can identify anomalies and patterns indicative of money laundering. These models learn from new data, making them highly adaptable to emerging threats.
For banks, generative AI-powered AML practices result in more accurate detection of illicit activities, reduced false positives, and enhanced compliance with regulatory requirements. Banks can safeguard their reputation, avoid hefty fines, and maintain trust with both customers and regulatory authorities.
Benefits and Challenges
Implementing Generative AI in banking brings forth a host of benefits and, in tandem, some challenges that require careful consideration.
Benefits:
- Improved Efficiency: Generative AI automates complex tasks, reducing manual labor and speeding up processes. This efficiency allows banks to handle tasks like credit risk assessment, customer service, and fraud detection more swiftly and accurately.
- Enhanced Customer Satisfaction: AI-driven chatbots offer round-the-clock support, leading to quicker responses and better service availability. Personalized marketing and wealth management solutions also result in higher customer satisfaction, as clients receive tailored recommendations and strategies.
- Better Risk Management: Generative AI helps banks identify potential risks more accurately. Credit risk assessments and fraud detection benefit from the precision and adaptability of AI models, reducing financial losses and maintaining the institution's credibility.
- Cost Reduction: Automation of routine tasks and streamlined operations lead to cost savings for banks. Additionally, improved risk management minimizes the financial impact of fraud and defaults.
Challenges:
Given that gen AI is still a relatively new approach to banking, it does bring with it its own set of challenges that cannot be overlooked.
- Data Privacy Concerns: Generative AI relies heavily on data, and with great data comes great responsibility. Banks must ensure the security and privacy of customer data. Mismanagement can lead to data breaches and tarnished reputations.
- Regulatory Compliance: The banking sector operates under stringent regulations, and compliance is non-negotiable. Integrating AI solutions necessitates careful adherence to regulatory requirements, such as GDPR, Basel III, and AML laws. Non-compliance can result in hefty fines and legal repercussions.
- Bias and Fairness: AI models can inherit biases from the data they are trained on. In banking, this can lead to discrimination in lending or other financial services. Ensuring fairness and transparency in AI models is a challenge that requires continuous monitoring and improvement.
- Adoption and Change Management: Integrating generative AI into existing banking systems can be complex. Staff training, change management, and ensuring that the AI complements rather than disrupts established processes are challenges banks must overcome.
Balancing these benefits and challenges is essential for banks looking to leverage generative AI effectively. By addressing data privacy, regulatory compliance, fairness, and change management, financial institutions can harness the power of AI while safeguarding their reputation and operations.
Getting Started with Generative AI in Banking
Generative AI is a strategic imperative in banking that can help completely transform the industry. In this blog, we've explored diverse use cases that demonstrate its power to enhance operations and elevate customer experiences. From risk assessments to personalized marketing, the benefits are clear: improved efficiency, higher customer satisfaction, and stronger risk management.
As we look ahead, the transformative potential of Generative AI remains boundless. Emerging trends like AI-powered financial advisors and predictive analytics are reshaping the industry. By embracing Generative AI and addressing its challenges, banks can lead innovation and deliver exceptional value. It's a journey towards a more efficient, secure, and customer-centric industry. Here at Ideas2IT, we offer Generative AI solutions tailored to the banking and financial sectors. If you want to take your financial business to the next level, we can help.
If you are looking forward to taking your banking services to the next level, feel free to contact us today! We look forward to learning more about your business and matching it with the right Generative AI Solutions!”