The evolution of Machine learning and big data has impacted all processes of every market segment. IT operations is one of the several processes. Big Data analysis in IT operations has helped teams optimize their IT processes through data-based decision-making and predicting potential issues. This is done through monitoring systems and gathering, interpreting, processing, and analyzing data from various IT operation sources. The process of streamlining IT operations through Big Data analysis is called IT Operations Analytics (ITOA).IT Operations Analytics (ITOA) enables you to eradicate traditional data silos in IT Operations by replacing them with Big Data principles. With ITOA, you could support proactive and data-driven IT Operations Management (ITOM) with clear and contextualized operational intelligence.As defined by TechTarget,“IT operations analytics (ITOA) is the practice of monitoring systems and gathering, processing, analyzing and interpreting data from various IT operations sources to guide decisions and predict potential issues.”There are several ITOA techniques. But the base ideology of all remains the same. Data from various IT operations are analyzed and used to project a high-level view of the entire infrastructure. This helps leaders to enable better management of IT resources, and employees and thereby build better infrastructure.
Why IT Operations Analytics (ITOA)?
In the past decade, we have seen IT operations transition from a tool-driven ideology towards a data-driven ideology. This is the origin story of Big Data in IT.In a tool-driven infrastructure, IT operations are implemented through a bunch of disconnected tools. All these tools will have independent records and data which are incompatible with the records and data from the rest of the tools. This results in separate islands of data that cannot be analyzed to get the bigger picture of the processes. With no way of analyzing the processes, it was impossible to trace bottlenecks and faults or predict potential issues or locate weak links.So, it became essential for all IT tools to be data-driven if the leaders wanted to analyze their IT operations. This led to the introduction of Big Data in IT operations. ITOA enables better performance, availability, and security analysis and helps leaders make more informed investment decisions. Additionally, to keep up with the ongoing changes and increasing competition, IT Operations and Management companies need to leverage advanced data science and machine learning.
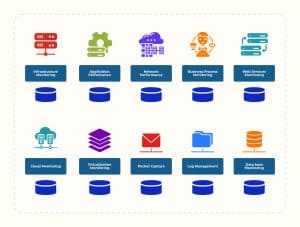
In 2017, the average cost of downtime was $100,000 for every hour of downtime on their site. For example, in 2017, a failure at British Airways resulted in a $102 million loss. - ForbesAs businesses start to implement automation of their own, I&O leaders will need to invest in “heuristic” capabilities that capture human learning and automate it. -GartnerBy 2019, 25% of global enterprises will have strategically implemented an AIOps platform supporting two or more major IT operations functions - Gartner
What problems does IT Operations Analytics (ITOA) solve?
You cannot manage what you cannot see and you cannot see the big picture if you are focused on one technology at a time. Some of the common issues faced by IT management companies are listed below:
Performance Problems
At certain high-traffic time periods, for example after advertising campaigns, or during pre-Christmas seasons, IT incidents cause poor performance leading to abandoned carts, dissatisfied users, and lost revenue.
Unresolved Issues
IT teams use a multitude of point solutions that do not share information. Correlating incidents is difficult causing alert fatigue and resulting in many incidents being left unresolved, increasing the likelihood for more such issues and costly downtime.
Time-intensive Error correction and RCA
When an error occurs in the system, it takes time for the root cause to be identified. This results in long error resolution times and dissatisfaction among users.
Slow Response Time
Any outage or problem needs to traverse the process of incident identification, logging, categorization, prioritization, diagnosis, and escalation to level 2 support before being resolved. This leads to slow response time, especially, in case of issues of smaller magnitude.
Best ITOA Features for Optimizing IT Operations
ITOA is not just about collecting data, how the collected data is analyzed and interpreted makes all the difference. An intelligent Data Analytics tool can help you with your ITOA needs. But before you choose ITOA tools, here are some of the features of an Intelligent ITOA (IT Operation Analytics) platform:
Incident Correlation
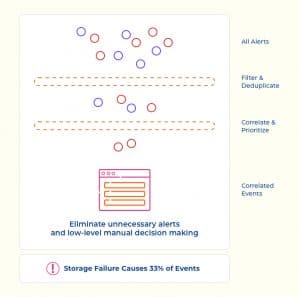
Your ITOA tool needs to have incident correlation abilities so that it can Intelligently cluster and correlate all the IT alerts into high-level incidents. So that you can focus on what is most important for your business. An efficient incident correlation feature:
- Correlates SMF log data with events and service models, or application groups while minimizing the need to manually define, configure, and maintain correlation rules and policies.
- Uses a standard event format to relate similar events, deduplicate and correlate with high accuracy.
- Uses proactive monitoring methodologies that can correlate alerts, and group-related events into parent-child relationships, and eliminate false alerts reducing the incident and alert fatigue.
- Uses text mining methods such as Latent Semantic Analysis (LSA), topic modeling, Bayesian classification, and clustering techniques to examine unstructured ticket data and classify/cluster them into problem patterns of groups.
- Converts tons of ticket data into numbered topics, function areas, and problem patterns that can be easily comprehended by SMEs and support and operation teams.
Incident Prediction & Scripting
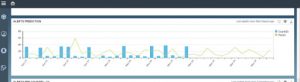
This feature applies Machine Learning to real-time data to analyze and predict anomalies even before they occur, hence reducing the Mean Time to Detect (MTTD). It also automates the execution of scripts preventing issues from occurring. Prediction and scripting of incidents:
- Analyses log data, metrics, events, changes, and incidents to predict anomalies within a single system and across systems.
- Automates cross-domain collection and indexing of logs and other machine data.
- Uses behavioral learning to search for anomalies and identify patterns and deviations.
- Uses an algorithm based on Robust Principal Component Analysis (RPCA).
- Creates proactive alerts when pattern deviations are detected.
- Forecasts future system states and any possible failures such as when a hard disk will be full? Which hard disk will fail? And more.
- Projects frequency of occurrence of repeated issues assisting IT Ops managers to detect, diagnose and resolve issues quickly and staff resources accordingly.
- Uses time-series forecasting to predict critical events and detect outliers to trigger alerts and execute scripts in advance preventing issues before they occur.
- Can execute a wide variety of actions such as restarting a struggling virtual machine, to adding more disk space so an application doesn’t exceed its quota.
Incident Agent Routing
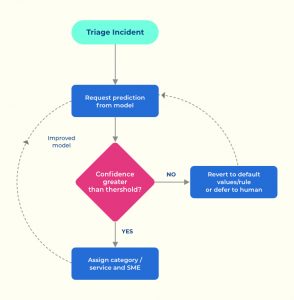
The Incident Agent Routing feature utilizes Artificial Intelligence services to determine which incident needs to be routed to which SME. It continuously learns from the routing process and automated assignment to improve the success rate. It can:
- Help reduce MTTR for incidents, and improve first-time resolution and user satisfaction.
- Automatically assign tickets without rule setting.
- The AI core analyses past incidents, alerts, and resolution routes to accurately determine key attributes with patterns of correlation to categories.
- Utilize past routing data to determine the subject matter experts who resolve certain categories or subcategories of tickets and automatically assign the ticket.
- Continuously learn from predicted assignments and new ticket routing data, to improve its assignment and success ratio.
Incident Scoring
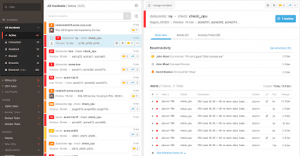
This feature also utilizes Artificial Intelligence. It records all the problems and determines what problems are important and need to be fixed first by allocating a priority score for all problems. Its additional functionalities are as follows:
- Shows a critical numerical score associated with each event/incident.
- Performs real-time scoring based on events, metrics, and log files in combination with ITSM processes such as CMDB, etc.
- Uses context analysis (including CI attachments, affected business services, etc.) and predictive forecast derived from historical data.
- Total score rolls upto to the incident level allowing identification of events and incidents which pose the highest threat.
- Maintains all source data when normalizing events allowing you to drill down to see where the scoring comes from.
- Prioritizes and automatically assigns high score incidents to relevant personnel based on the assignment of past tickets.
Incident Resolution
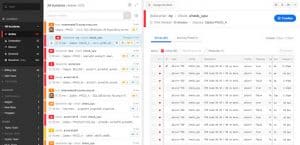
The Incident resolution process applies Machine Learning to accelerate the resolution of incidents by contextualizing information: for e.g. linking related tickets, people, knowledge base articles, and suggesting resolutions where possible. The features are listed below.
- Uses blended analytics to find relevant, contextual, and time-sensitive data.
- Provides cross-silo view and insights by assimilating and normalizing changes and incidents with log files, time-series data, and events and linking incidents with related tickets.
- Uses text mining such as LDA to connect and categorize and connect events, tickets, knowledge base articles, alerts, and changes using a cause-effect relationship
- Automatically assigns high-priority tickets to service personnel based on trends in historical data.
- Identifies batch load to understand application performance and perform a batch job analysis.
- Dynamically identifies batch execution patterns using machine learning algorithms for batch job analysis.
- Allows identification of any deviations in batch runs allowing pre-emptive fix.
Root Cause Analysis
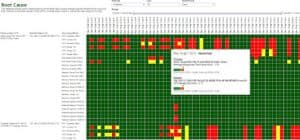
ITOA leverages Machine Learning and leverages knowledge of experts, by analyzing logs and all past changes, performing pattern recognition and statistical modeling to identify the potential root causes. This can be extended to cover incidents, problems, changes, and configuration management. Apart from identifying the root cause of all the issues, the root cause analysis feature also:
- Collects detailed logs and diagnostic data from every monitored application.
- Tracks changes in various dimensions: from capacity issues to shifts in workload sequence or volume to changes in code for root cause analysis.
- Detects differences between working and non-working environments, using environment comparison. It also allows you to define diagnostics for KPIs and trigger actions to collect additional input and automate workflows, such as checking for recent changes in CMS.
- Uses probable cause analysis for root cause analysis.
- Ranks and scores probable causes using machine learning, historical probability, CMDB relationships, and temporal alignment.
- Allows you to eliminate a huge share or number of problems using Pareto’s concept and diagnose intermittent issues on demand.
- Has been seen to reduce MTTF by 75%
Getting Started with IT Operations Analytics (ITOA)
In order to execute a typical ITOA Project based on a procedure-based model, you can build the model in multiple stages. Below are the various stages in which we suggest you create your ITOA system along with the expected deliverables in every stage.Stage 1: Define Strategy & Goals
- Define project goal/problem statement
- Define Stakeholders
- Define project risks
- Define project plan
Stage 2: Analysis & Design
- Overview of current infrastructure and design
- Design of an ITOA system architecture
- Define the authorization concept
- Identify and explain relevant data sources
Stage 3: Implement & Connect Data Sources
- Install and configure the ITOA system
- Connect internal data sources & third-party systems
- Data cleansing and definition of data fields for analysis
Stage 4: Data Analysis
- Define search queries to answer from the strategy phase
- Identify the use of the extension for reports
- Integrate validated search queries into dashboards and reports
Stage 5: Modelling & Evaluation
- Validate statistical or data mining models
- Forecasts for capacity bottlenecks, new relationships that cause malfunctions in infrastructure
- Implemented models in ITOA systems that analyze incoming data continuously
Stage 6: Optimization & Transformation
- Recommend actions based on analysis
- Recommended implementation of next maturity level
- Train users to work with the IT operations Analytics system
Conclusion
To further improve your IT processes, you can consider adopting IT Process Automation (ITPA) practices with ITOA. ITPA utilizes the data analysis and interpretations from ITOA systems and helps in the automation of IT processes.The aim is to:Automate everything that can be automated.Optimize the rest to eventually automate it.