IntroductionA lot of valuable insights are logged in enterprises' huge corpus of documents. Retrieving the right insight for the right context is extremely important in unlocking this value. For instance, getting answers to a question posted via a chatbot greatly depends on unlocking the answer from the appropriate document.Commonly applied full-text search often doesn’t return compelling answers. In this blog, we will explore Natural Language Processing based Answering System for information retrieval.It can be achieved through both supervised and unsupervised techniques. What we have created is an Unsupervised Information Retrieval System, DocSearch which can be integrated into a chatbot as a service where the user can get information through natural queries.
Understanding What Is Docsearch?
Docsearch system is a software-based framework designed to retrieve relevant information efficiently and effectively from a collection of data or documents in response to user queries.
These systems are integral to various applications, such as search engines, recommendation systems, document management systems, and chatbots. The primary goal of a Docsearch system is to bridge the gap between the user’s information needs and the available data by providing timely and accurate results.
Unlike simple keyword-based searches, modern Docsearch systems employ advanced techniques from fields like Natural Language Processing (NLP), machine learning, and data mining to understand user intent, context, and the semantics of queries and documents. This enables them to retrieve documents that match the exact keyword and answer the user’s query.
Information Retrieval Process Using Docsearch
Docsearch, powered by advanced natural language processing (NLP), offers a streamlined approach to finding relevant information from vast document repositories. Here’s a look at the information retrieval process using Docsearch.
Data Ingestion
The first step involves collecting and ingesting documents into the system. Docsearch supports various formats, ensuring that all relevant data sources are included. This foundational stage is critical for building a comprehensive search index.
Indexing
Once documents are ingested, Docsearch processes and indexes the content. This involves parsing the text, extracting keywords, and creating an efficient structure that allows for quick retrieval. The indexing process enhances search speed and relevance.
Query Processing
When a user submits a query, Docsearch utilizes NLP techniques to understand the intent behind the question. This step involves tokenization, stemming, and identifying key phrases to accurately interpret the user’s request.
Retrieval and Ranking
After processing the query, Docsearch retrieves relevant documents from the indexed database. It ranks the results based on various factors, including keyword relevance, context, and document quality, ensuring that users receive the most pertinent information.
Response Generation
Once the relevant documents are identified, Docsearch formulates a response. This may include snippets from the documents, direct answers to questions, or links to full documents, depending on the user’s needs.
User Feedback and Continuous Improvement
To enhance the accuracy and relevance of future searches, Docsearch can incorporate user feedback. This iterative process allows the system to learn from interactions and continuously improve its performance over time.
Getting the Data
The data can be from multiple sources like web repository, PDF, word or text documents. All the documents are scraped, preprocessed and collated into a corpus in S3, which acts as a single source of information for model creation.
Model
We used Latent Semantic Indexing (LSI) to find and rank the documents. LSI model is one of the Information retrieval methods. It is based on the Distributional hypothesis ie., words that occur in the same contexts and tend to have a similar meaning. It helps in producing concepts related to the documents. We can use this to find similarities in the documents.

Developing LSI Model
Create a matrix of term frequency in respective documents, representing the number of times the term appears in the document. The matrix is large and sparse.
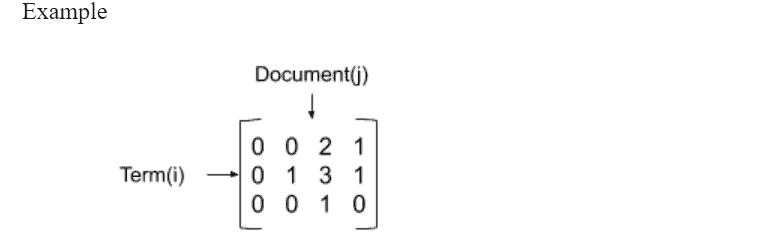
Each cell represents the frequency of term i in document j.
- On the Term-Document matrix, apply a weighting function. The weighting function transforms each cell to weight relative to the frequency of a term in a document and the frequency of the term in the entire document collection. Here we have used the Log-Entropy weighting function, similar to TF-IDF.
- Perform a low-rank approximation to reduce the dimension of the matrix. In LSI, Singular Value Decomposition(SVD) is used to reduce the dimension.
- After the decomposition of X, an mxn matrix, where m is the number of unique terms and n is the number of documents, we get below three matrices.
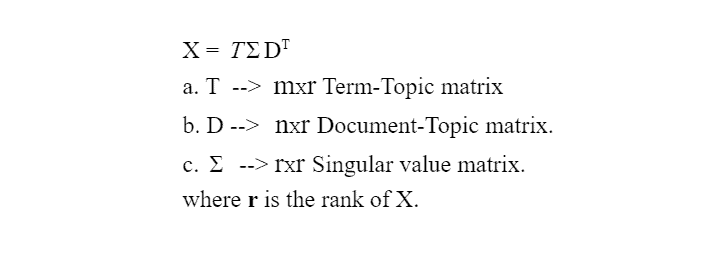
- The SVD is truncated to reduce the rank to k << r, where k is typically in the order of 100 to 300. In our implementation, we chose k as 200. Finally,
- -> Tk is a matrix of term vectors
- -> Dk is a matrix of document vectors
The Document-Topic matrix(Dk) which creates a document vector space, helps us in finding the documents relevant to the query.
Ranking Documents
- When the query comes in, the sparse term frequency vector(q) is computed and the weighting function is applied. Then the sparse vector will be converted into a dense query vector(Qk) in document vector space, using the below equation.
Qk = qTTk-1
- The similarities between the query vector (Qk) and the document vector (Dk) is calculated using Cosine similarity.
- Based on the similarities we can rank the documents and show the user the best matching documents.
Model improvements
- We have implemented domain-specific preprocessing to improve the quality of the document corpus for the model.Eg. Normalising Volt, voltages, V to volt
- Instead of a unigram model, we implemented a bigram model to increase the relevancy. The bigram model converts all unigram words to bigram and helps in finding documents with appropriate phrases.Example,In the unigram model: ‘safety’, and ‘switch’ will be the search word that gives noisy results.In the bigram model: ‘safety switch’ will be the search word that will produce relevant results
Query Modification
- Query alteration - We implemented a generalized HMM model to correct the errors of the query, like spelling mistakes, merging errors, splitting errors,s and misuse.
- Query expansion - An external domain-specific synonym mapper helps to expand the query with the synonyms, which helps in getting more relevant documents.

Model Updating
Whenever a new document is uploaded the model will be updated with the new documents and the entire flow has been automated.
conclusion
In the world of Natural Language Processing, DocSearch stands out by revolutionizing how we interact with vast data repositories. By seamlessly connecting user queries to relevant information, it enhances the efficiency and accuracy of data retrieval.
As AI continues to evolve, grasping the interplay between Information Retrieval and Natural Language Understanding becomes essential for harnessing the full potential of intelligent answering systems. Embracing solutions like DocSearch is vital for navigating the complexities of modern information landscapes.